Google makes privacy-focused data analysis tool open source
Its differential privacy library has helped shape many of the company's core products
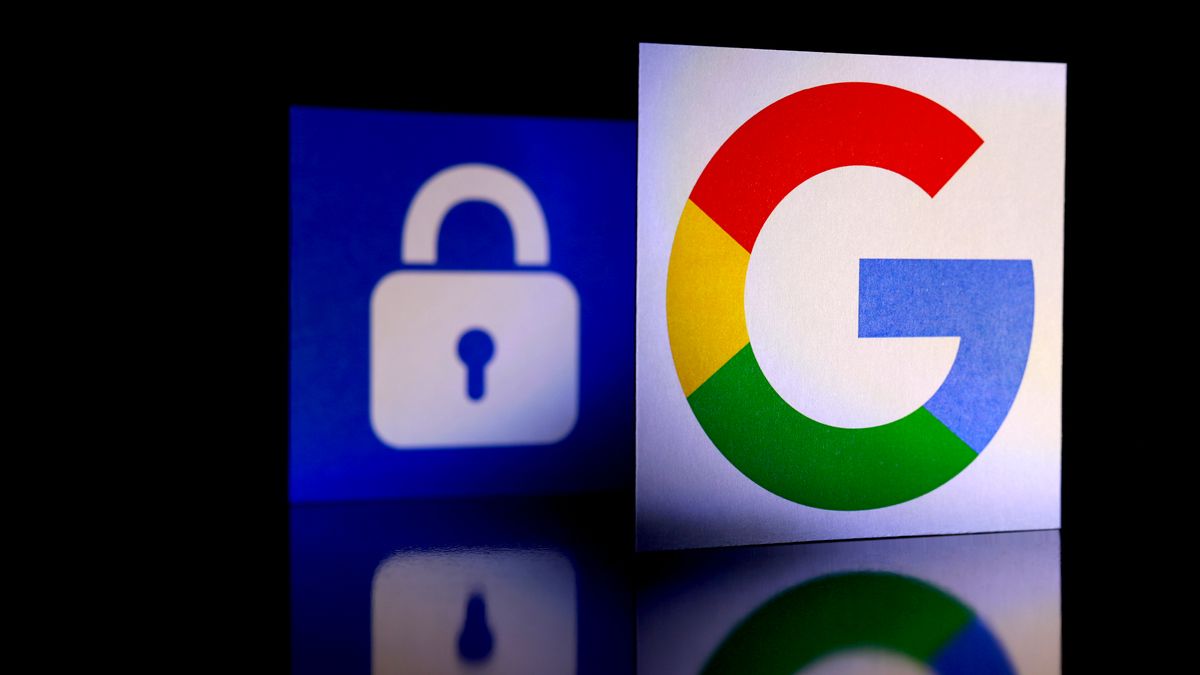
Google is launching an open source version of its internally used differential privacy library, allowing businesses and data scientists to generate insights from data while protecting the privacy of those to which it belongs.
Google's differential privacy library is used to make improvements to many of its core products, such as when Search knows how busy a business, such as a gym, is at certain times or how popular a dish is at a given restaurant.
Differential privacy is an approach to data science which involves taking large amounts of user data and obfuscating it with artificial data - enough to hide a user's true identity but not so much that insights can't be made using software-aided analysis.
Businesses can now use Google's library to start forming their own conclusions from big datasets without their customers losing trust in their brand, the company argues.
In addition to Search, Google has embedded differential privacy in products since 2014. RAPPOR (Randomised Aggregatable Privacy-Preserving Ordinal Response) was a Chrome privacy project designed to better safeguard users' security, find bugs, and improve the overall user experience while analysing user data.
Adding to the growing list of privacy-minded applications, TensorFlow privacy was introduced this year to help protect users from being identified when their data was being used to train AI algorithms.
Apple is another company that's been hot on embedding differential privacy into its work. Since 2016, the privacy mechanism has been used in its machine learning algorithms to analyse the plethora of data it takes from its customers' iPhones.
Get the ITPro. daily newsletter
Receive our latest news, industry updates, featured resources and more. Sign up today to receive our FREE report on AI cyber crime & security - newly updated for 2024.
Data is becoming increasingly valuable, some experts even say its the most valuable commodity in the world and it's something that hackers can steal and sell on for profit.
In a world where data breaches are rife, protecting data and the user to whom it belongs can be a hugely significant factor when it comes to maintaining customer trust.
Unfortunately, not every company gets it right - even the big names. In the late 2000s, well-meaning Netflix aimed to improve its film recommendation algorithm by using supposedly de-anonymised data which eventually was found to not be sufficiently protected.
Researchers were able to reveal user identities form the large dataset and even pinpoint their political affiliation.
"This sort of thing should be worrying to us," said Matthew Green, cryptography professor at Johns Hopkins University in a blog post.
"Not just because companies routinely share data (though they do) but because breaches happen, and because even statistics about a dataset can sometimes leak information about the individual records used to compute it," he added. "Differential Privacy is a set of tools that was designed to address this problem."
One real-world benefit of a differential privacy approach relates to health research, as explained by Miguel Guevara, product manager, privacy and data protection office at Google.
"If you are a health researcher, you may want to compare the average amount of time patients remain admitted across various hospitals in order to determine if there are differences in care," he said.
"Differential privacy is a high-assurance, analytic means of ensuring that use cases like this are addressed in a privacy-preserving manner."
Connor Jones has been at the forefront of global cyber security news coverage for the past few years, breaking developments on major stories such as LockBit’s ransomware attack on Royal Mail International, and many others. He has also made sporadic appearances on the ITPro Podcast discussing topics from home desk setups all the way to hacking systems using prosthetic limbs. He has a master’s degree in Magazine Journalism from the University of Sheffield, and has previously written for the likes of Red Bull Esports and UNILAD tech during his career that started in 2015.