Five steps to big data project success
How organisations can get an end-to-end view of their data pipelines
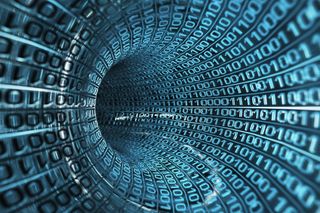
Big data has the potential to both create transformational business benefits and solve big problems. While a whole ecosystem of tools has sprung up around Hadoop to analyse and handle data, many are specialised to just one part of a larger process.
When companies leverage Hadoop effectively, the potential business and IT benefits can be especially large. But as with any technology just beginning to mature, high entry barriers can create challenges for successfully implementing Hadoop as a value-added analytics tool.
To make the most of Hadoop, businesses need to take a step back and have an end-to-end look at their analytics data pipelines.
1: Ensure a flexible and scalable approach to data ingestion
The first step in an enterprise data pipeline involves the source systems and raw data that will be ingested, blended and analysed. Combinations of diverse data initially isolated in silos across the organisation often lead to the most important big data insights.
Because of this, the ability to utilise a variety of data types, formats and sources is a key need in Hadoop data and analytics projects.
Not only should organisations prepare for the data they plan on integrating with Hadoop today, but also the data that will need to be handled for other possible use cases in the future. Planning to reduce manual effort and establishing a reusable and dynamic data ingestion workflow are vital parts of this.
2: Drive data processing and blending at scale
Once enterprises can successfully pull a variety of data into Hadoop in a flexible and scalable fashion, the next step entails processing, transforming and blending that data on the Hadoop cluster at scale.
Get the ITPro. daily newsletter
Receive our latest news, industry updates, featured resources and more. Sign up today to receive our FREE report on AI cyber crime & security - newly updated for 2024.
There must also be a level of abstraction away from the underlying framework, whether that be Hadoop or something else, so the maintenance and development of data-intensive applications can be democratised beyond a small group of expert coders.
In a rapidly evolving big data world, IT departments also need to maintain and design data transformations without having to worry about changes to the underlying structure. Instead of taking black box' approaches to data transformation on Hadoop, organisations should try for an approach that combines deeper control, visibility and ease of use.
Don’t just collect data, innovate with it.
Removing the barriers to the experience economy
3: Deliver complete big data analytic insights
Carefully considering all relevant business processes, applications and end-users that the project should touch is a prerequisite to unlocking maximum analytic value from Hadoop. Depending on what data they need, their plans for that data and how sophisticated they are, different end users may need varying approaches and tooling.
Advanced analysts and data scientists will often make use of data warehouse and SQL-like layers like Hive and Impala when they begin querying and exploring data sets in Hadoop. Luckily, these don't take long to learn because of the familiar query language.
High-performance and scalable NoSQL databases are increasingly being used in tandem with Hadoop. Operational big data gleaned from web, mobile and IoT workloads is structured in NoSQL architecture before being funnelled into Hadoop software. In return, batch and streaming analytical workloads processed by Hadoop can be shared with the NoSQL architecture.
The rise of NoSQL paired with the value of big data being revealed is causing organisations to seek IT professionals with both NoSQL and Hadoop skills to make the most of their big data.
Considering Hadoop as part of the broader analytic pipeline is crucial. Many businesses are already familiar with high-performance databases that are optimised for interactive end-user analytics, or analytic databases.' Enterprises have found that delivering refined datasets from Hadoop to these databases is a highly effective way to unleash the processing power of Hadoop.
4: Take a solution-oriented approach
While many advancements have been made in the Hadoop ecosystem over the past few years, it is still maturing for use in production enterprise deployments. Requirements for enterprise technology initiatives tend to evolve and be works in progress,' which is where Hadoop represents a major new element in the broader data pipeline. As a result of this, related initiatives normally require a phased approach.
With this in mind, software evaluators will not find one off-the-shelf tool that satisfies all current and forward-looking Hadoop data and analytics requirements. Without overdoing the term future-proofing,' extensibility and flexibility should be a key part of all project checklists.
The ability to port transformations to seamlessly run across different Hadoop distributions is a starting point, but true durability requires an overall platform approach to flexibility that aligns with the open innovation that has driven the Hadoop ecosystem.
Don’t just collect data, innovate with it.
Removing the barriers to the experience economy
5: Select the right vendor
The big data boom has resulted in a surge of solution providers flooding the market. The packages they offer can vary widely, ranging from simple statistical tools to advanced machine-learning applications.
Organisations should identify the data types they will be processing to select a technology that accommodates them. A desirable platform would also feed existing analytics tools, giving employees the access they need with minimal disruptions to workflow.
Some NoSQL and Hadoop providers are teaming up to provide a comprehensive offering, integrating their systems to streamline the flow between the architecture and the software. This also reduces complexity for customers, as they can deal with just one point of contact.