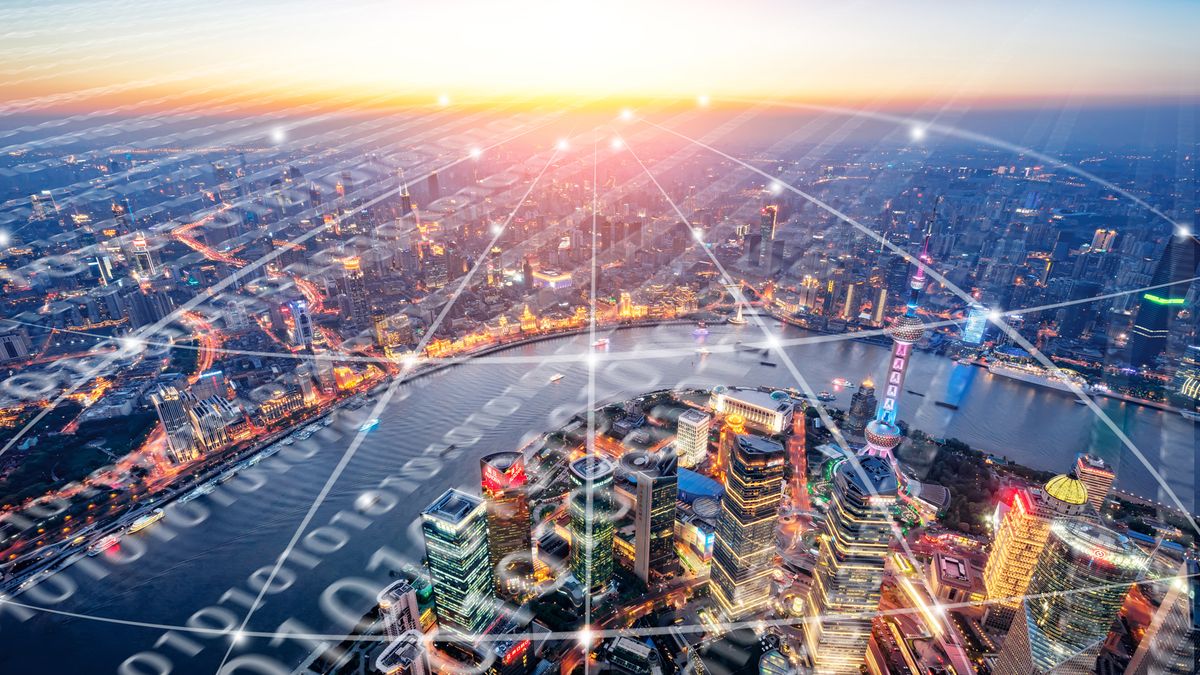
Businesses of all sizes are undergoing rapid digital transformation. Innovation and disruption are encouraging organisations to think ahead and position themselves for future challenges and opportunities. Underpinning all of this is data and the race to extract meaningful insight and gain a competitive edge. Growth is such that the world’s data is forecast to reach a staggering 175 zettabytes by 2025.
With volume surging, products that are powered by data or come from an extracted data set are proving to be invaluable business assets, yielding very high margins. Data products can be classified in many different ways, but the most common sit across three types: data as service, consisting of industry-specific information services (eg business contacts data, weather data); data powered products, which use data to help a product increase its value (think online search engines); and data as insights, such as marketing or consumer data insights.
Though these three categories may result in different data product outcomes, the building blocks for all are the same. Here we look at some of the essential questions that need to be asked when creating a successful data product.
What is the value proposition of the data product to the end user?
In building a data product it’s important to understand how that product will support the customer journey and increase value for the business. Once that is understood, it becomes easy to identify sources of the right data, how the data will add value to the client, how the product can be shaped around the data and how good feedback loops with the customer can be engineered. Moreover, the data can be useful in building models, creating a good UI or dashboards, and enhancing the user experience.
Gladys Christopher, director, data solutions at Merit, stresses the importance of having a “holistic understanding” of an organisation’s larger goals before embarking on a data product. Merit provides data collection, data transformation, bespoke software and business intelligence for some of the world’s leading B2B brands.
“At Merit we are able to power the world’s leading corporate brands as we not only understand our clients’ requirements and expectations but develop a holistic understanding of the wider goals, objectives and the final destination,” Christopher says. “We make it our business to partner and collaborate with the client in their customer journeys instead of merely having a vendor/supplier relationship. With every client we strive to transform and innovate deliveries, in a small or significant way, providing solutions that are better, quicker and cost effective.”
Are the right stakeholders involved?
Designing and building an effective data product requires collaboration between many people of varying skills and expertise. Crucially, it needs to bring together a group of people who will be able to understand customer journeys and bring maximum value to the end user. Data engineers, solution architects and developers will serve the technical side, while product managers and sales managers will have a grasp of the needs and requirements of users.
Are the right (and cost-effective) tools in place?
It’s always important to take a step back and establish what your overall objectives are and ask if the technology or tools you want to implement will help you hit that target. Will it deliver within the expected time frame or can it be done in a shorter duration? Many organisations jump into adopting new tech without thinking about both the direct and hidden costs of the new solution. For example, companies can often lay out significant expenditure on new tech because they don’t want to be seen using legacy solutions. Without proper staff training or expertise, this can result in the new investments being underused and legacy (and less effective) tech continuing in the organisation for longer than it should.
There are also cases where existing tools aren’t equipped for the job and it’s much more efficient to build bespoke data solutions. Some organisations view pre-built AI or machine learning-based data platforms or RPA solutions as the most cost-effective way to scrape data from all types of sources and all levels of volumes and complexities. Though data scraping might be a repetitive task, if you are looking at a varied and wide variety of sources it can make data scraping very challenging. This is especially true if the information is to be gleaned from unstructured data (reports, news, pdfs, documents, etc) as well as more structured data points (such as product name, description, price, etc). Collecting data at a large scale can accentuate that complexity; clunky database handling, high infrastructure costs and blocked data collection requests can collapse moderate or high volume data collection. In this instance, a custom solution will get you to your goal faster. Many organisations think that anyone can use self serving data platforms and get the results required. However, there are very few cases where a non-tech person can execute these solutions – almost all purchased or subscription-based data platforms require further investment in manual tech resources, meaning there can be significant indirect costs attached.
The last thing organisations want is to end up paying additional costs to make the solution work, thus removing any cost efficiency from the endeavour.
Do you have the right data sets?
Having timely, comprehensive and accurate data will always bring the best results. Missed data points, duplicated content or delayed information will quickly undermine confidence in the product or service. Even the most brilliant data scientists and engineers won’t be able to build a good product if the underlying data quality is poor. Time invested in refining or transforming raw data – cleansing, normalising and standardising – is time well spent. If the data product being built is a solution to a data problem, having huge quantities of data is of no use to even the most brilliant AI developer if the quality is not aligned with the problem they are trying to solve. Where end users spot gaps or deficiencies in the data, their confidence and willingness to engage with the product will dissipate quickly.
In addition to that, sometimes having small quantities of good quality data isn’t enough, particularly if you are building predictive models – you need large quantities of data from disparate sources to reduce false positives. A good balance between quantity and quality of often key to building accurate data models.
Is the future objective your current priority?
Data products increase in value the more data they collect, and can provide powerful inputs for the improvement or creation of other new data products. Feedback loops or inbuilt algorithms that feed the results of the user interactions back to the product are key. For example, let’s say the data product provides regulatory content from various sources. It is extremely useful to compel the user to record the decision on what type of content (from which source) is relevant. Feeding the results back and improving the ML is a good way to eliminate false positives from the source before the end user sees it, which in turn will save valuable time. Investing time in building an iterative feedback loop within your data product should not be optional or postponed but an integral part of your creation process.
One final point to keep in mind is that a data product will not give 100% accuracy, especially at the early stages, unless input is static or the decision making is rule-based. However, data products can be fine-tuned to a high level of accuracy using a combination of AI or machine learning algorithms and the feedback from the user interactions of the system over a period of time.
Discover more about data products at meritgroup.co.uk
Get the ITPro. daily newsletter
Receive our latest news, industry updates, featured resources and more. Sign up today to receive our FREE report on AI cyber crime & security - newly updated for 2024.
ITPro is a global business technology website providing the latest news, analysis, and business insight for IT decision-makers. Whether it's cyber security, cloud computing, IT infrastructure, or business strategy, we aim to equip leaders with the data they need to make informed IT investments.
For regular updates delivered to your inbox and social feeds, be sure to sign up to our daily newsletter and follow on us LinkedIn and Twitter.