What is 'dark data' and how could it be impacting your cloud migration?
Too many businesses are tackling the problem of dark data with additional storage, but this is an expensive and inefficient fix
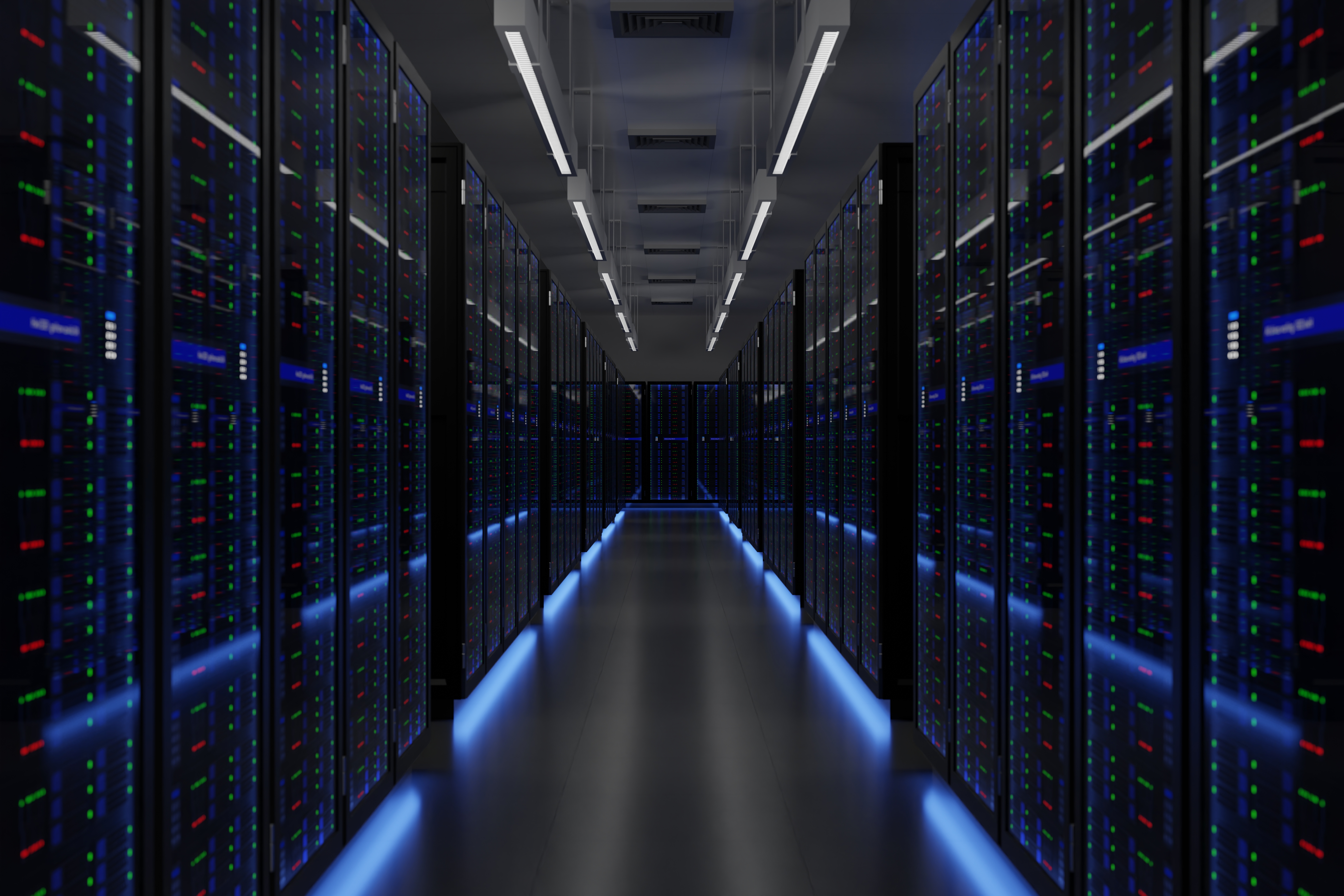
Dark data has become something of a theme among IT professionals in recent years, seemingly offering the ability to upgrade the way data is collected, handled, and stored within large organisations and improving operations as a result.
As companies move more of their data to the cloud – or, more accurately, to managed data centers and on premise storage – the ability and willingness to gain insights into almost everything via that data grows, and becomes attractive as a strategy to maximise business advantages over competitors, and improve operations.
What is dark data?
Gartner defines data data as "the information assets organizations collect, process, and store during regular business activities, but generally fail to use for other purposes (for example, analytics, business relationships and direct monetizing)".
"Similar to dark matter in physics, dark data often comprises most organizations’ universe of information assets. Thus, organizations often retain dark data for compliance purposes only. Storing and securing data typically incurs more expense (and sometimes greater risk) than value."
We put this definition to David Hand, an emeritus professor of mathematics and senior research investigator at Imperial College London, author of Dark Data: Why What You Don’t Know Matters. Although he submits the definition is correct to a degree, he argues that "it’s very narrow and completely misses the most important types of dark data".
He argues that the phenomenon is better defined as "data you don’t have", which "may be data you wish you had, or hoped to have, or thought you had, but it is nonetheless data you don’t have". So rather than just data that has been collected, dark data can be applied much more broadly.
Instead of a narrow definition, Hand suggests there are 15 kinds of dark data (although there is some overlap between them), including data we know is missing, data we don’t know is missing, choosing just some cases, self-selection, missing variables, data which might have been, and measurement error and uncertainty.
Get the ITPro daily newsletter
Sign up today and you will receive a free copy of our Future Focus 2025 report - the leading guidance on AI, cybersecurity and other IT challenges as per 700+ senior executives
Hand says Gartner’s definition is the third example, 'choosing just some cases', or "data which has been collected but which is then being ignored". In some senses, this type of data is one of the easiest to deal with as it exists.
Where is dark data?
According to estimates from Veritas, almost half of the information stored on the secondary data stores used for migration to the cloud is dark; unlabelled data that could potentially land the organisation in hot water with government regulators.
But that’s only if the data has actually been collected in the first place. "You may know you have not collected it, or you may not know," says Hand. "A familiar example of the first would be missing values in a data table and a familiar example of the second would be missing responses in web surveys, where you may not have a well-defined population so you don’t even know who hasn’t replied."
In effect, "the most significant cost of dark data arises from the mistaken conclusions and decisions you are making because of failure to understand dark data"; the answer to "where is dark data?" could easily be "nowhere" for some companies.
The negative effects of dark data
While the term "dark data" might have somewhat negative connotations, the simple fact of considering its very existence is a positive step for many companies. As the old adage goes, you don’t know what you don’t know. There are, of course, still downsides.
One of the most serious adverse effects of dark data is "business decisions made in good faith on the basis of inadequate data", according to Hand, which is also something that could be avoided. In fact, the dark data expert says that many data science courses "do not treat the topic of dark data adequately". It's possible for companies to realise their mistakes, but oftentimes this happens after operational decisions have been made with inadequate data to back them up.
Beyond financial costs, dark data can also present compliance and security risks. Since it’s often unclassified or forgotten, dark data can contain sensitive information that remains unprotected. As a result, businesses might fail to meet regulations like GDPR, which mandates the secure storage of personal data. In a worst-case scenario, this can lead to costly fines and reputational damage.
When migrating to the cloud, the presence of outdated or irrelevant data can complicate cloud strategies. IT teams waste time sifting through irrelevant files, leading to project delays and increased complexity. Additionally, unstructured data can skew analytics, producing misleading insights that undermine decision-making.
How to deal with dark data
"The first step must always be to be aware there might be dark data," argues Hand. "Indeed, your default assumption should be that the data are incomplete or inaccurate." For him, that is the most important message: "be suspicious about the data — at least until it is proved they are adequate and accurate."
"Additionally, you need to be able to recognize situations especially vulnerable to problems of dark data, particular signs that invisible dark data are distorting what has been collected, and more general situations in which danger lurks." No one said running a business was easy, after all.
To mitigate some of these challenges, businesses should conduct a comprehensive cloud audit before a migration, identifying and classifying data to determine what should be retained. Implementing data governance policies ensures that new data is adequately managed, while archiving or securely deleting dark data can prevent further accumulation.
Addressing dark data is essential for a smooth and cost-effective cloud migration. With careful planning and data management, organizations can avoid the pitfalls of transporting unnecessary information and instead build a leaner, more agile data infrastructure.
-
SAS wants its AI agents to supercharge workers, not replace them
SAS has announced a new agentic AI service aimed at helping enterprises deploy agents alongside domain-specific AI models.
-
SAS leans on synthetic data and digital twins to support business data demand
News SAS has announced new synthetic data capabilities and support for digital twins in a bid to equip enterprises with concrete platforms for data-driven decision making.
-
Power supply shortages are a ticking time bomb for data center operators
News Two-fifths (40%) of data centers will face constraints in power availability by 2027.
-
Looking for profit in the Internet of Things
In-depth Inside the Enterprise: How can CIOs make the most of the opportunities presented by the Internet of Things?
-
Internet of Things early adopters encouraged by Gartner
News Gartner claims IT leaders that get to grips with IoT early will be best placed to take advantage of emerging business opportunities.
-
Apple on top in smartphone wars
News The iPhone maker led the pack in 2011, thanks to record sales of its mobile device.
-
Apple and Android gain share as Nokia falls
News Nokia's market share falls to its lowest since 1997, as Apple and Android sees notable gains.
-
Week in Review: Nokia updates Symbian, browser wars kick off
News This week, Symbian gets Nokia's attention whilst the browser giants are at it again.
-
Mobile sales soar as Nokia share falls
News Nokia is losing more market share, as mobile and smartphone sales rise in 2010.
-
Nokia profits fall
News The Finnish phone maker sees profits fall as the new CEO calls for changes.