Make better decisions with analytics
Analytics can transform a business, but it takes the right data, processes and technology to work
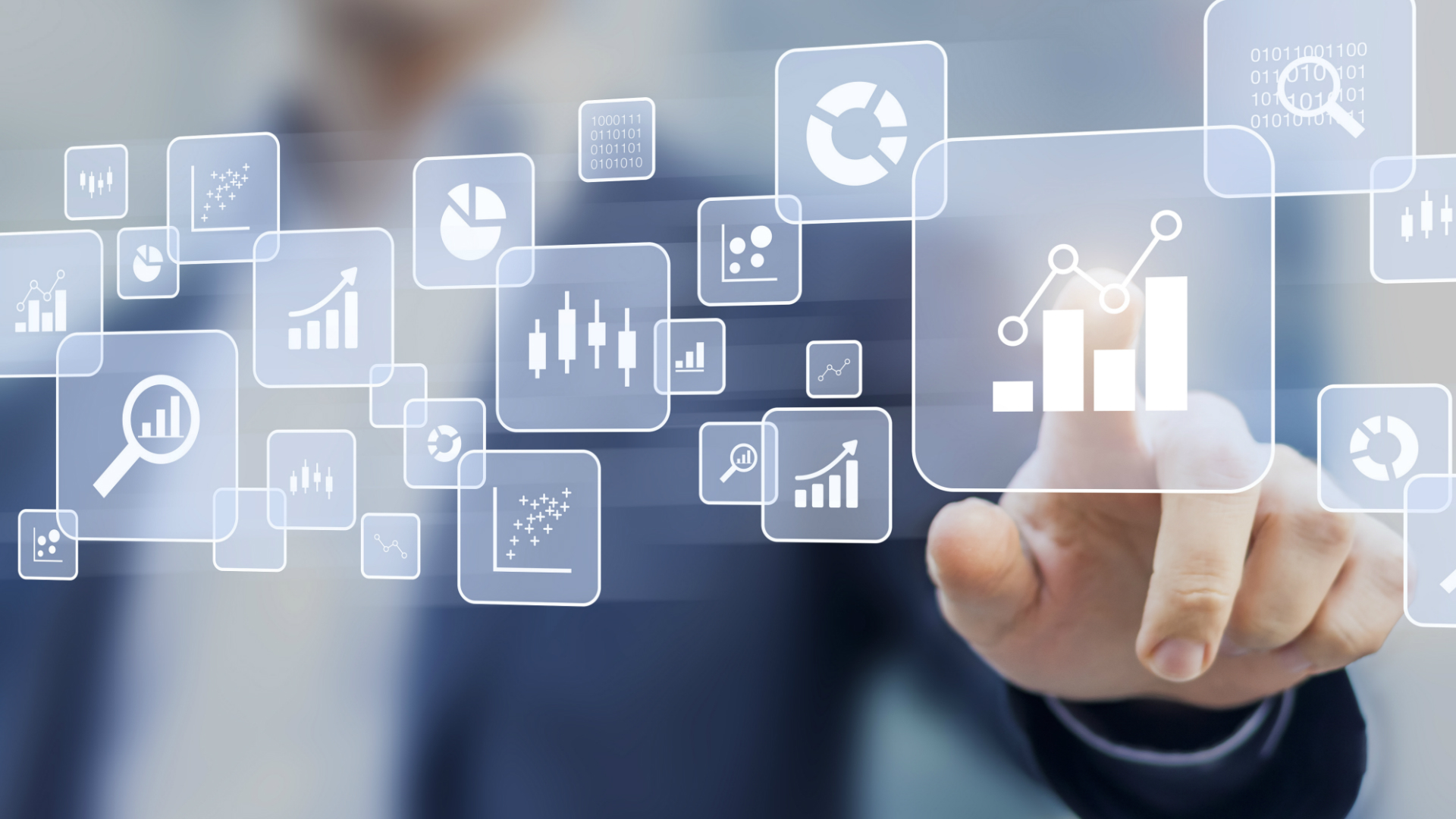
Data on its own can't drive business decisions; it can't help companies compete in challenging markets, push productivity or deliver a better service to its customers. Combine data with analytics, however, and it's a different story. Analytics applications can unlock the valuable insights hiding within all that data and most importantly turn them into something a company can use to take action, so that they can make their products stronger and more reliable, enhance their services and anticipate their customers' needs. In short, it's not having the data that counts, but what you do with it that matters.
This is crucial. A 2016 parliamentary report found that despite data driven companies being 10% more productive than those that do not operationalise their data, most companies estimate they are analysing just 12% of their data.' A 2016 report by Keystone Strategy for Microsoft found that companies who used data effectively outperformed their peers across a range of core business metrics, including profits, margins and employee productivity. At the same time, analysts at Gartner have claimed that anywhere between 60% and 95% of big data projects fail, primarily because companies find it hard to integrate analytics into their existing processes.
Combined with analytics and integrated into company processes, big data can be transformative. The UK insurance company, Markerstudy, saved millions and lowered customer cancellation rates by 50% by using Apache Hadoop and Spark to analyse hundreds of millions of quotes in seconds, then using Zoomdata to deliver those insights in easy-to-understand visualisations. It's not just beating its rivals on speed, but also on accuracy.
Transport for London is using data from Oyster card and contactless payments, along with bus location and ticketing data, to optimise its transport networks and minimise the impact of closures or diversions. Meanwhile, developers are using its real-time data streams to power hundreds of transport information apps.
Tesla has been a pioneer in in-car data collection, using data captured to spot, anticipate and predict potential problems before they can harm the firm's reputation, but it also ties this information into the data captured about its customers, so that it can target its resources to improve their experience. While other high-end manufacturers are responding to shifts in customer sentiment, Tesla is actively anticipating them and working out how to handle them.
Meanwhile the European clothing manufacturer and retailer, Zara, uses data captured in real-time on fashion trends and customer activity on the shop floor to work out what their customers are looking for and rush new designs from concept stage to retail within three weeks or less. Where less data-driven rivals have to gamble on a fashion trend or may take months to bring a key look into their lines, Zara can do it within 21 days.
Most UK supermarkets now use analytics to work out which products make the most profit in which locations in a given store layout, then use that information to drive sales. Many also use analytics to isolate promotions that can act as loss leaders plus the higher-value sales they'll drive.
From project to process
How, then, can you make sure you're putting your company's wealth of data to good use? It starts with preparation. Data needs to be broken out of company siloes, refined and combined into streams that analytics applications can use. It's not so much a question of separating the wheat from the chaff as getting some idea of what business challenges you're hoping to solve and which data sources will give you the most current, accurate and relevant information.
You then need to work out how and when to operate on that data. Early analytics projects focused on taking periodic snapshots in order to analyse what had happened, highlight trends and help companies plan for the future. Increasingly, though, companies are moving to systems that analyse incoming streams of data at a faster rate or even in real-time in order to spot patterns that predict what's most likely to happen and make active recommendations about how to take action.
This, however, entails a further stage; to bring these recommendations into the everyday business process in a form employees will actually understand and use. This may mean using the results in daily meetings or transforming insights into a narrative to follow, or increasingly it means creating dashboards with performance indicators, visualisations and automated data and recommendation feeds.
A recent McKinsey Report, Analytics Comes of Age, spoke to numerous executives at large enterprises. A CEO of an investment bank suggests that "by relying on the statistical information rather than a gut feeling, you allow the data to lead you to be in the right place at the right time". Another executive describes how their sales teams resisted using leads generated by the analytics model until these recommendations were integrated into daily workflow dashboards, so that they were simply presented as leads to follow. When analytics become part of the daily workflow, so do the results.
In the end, this means cultivating a data-driven culture, where insight, not gut-feeling, drives things forwards, and where companies can move faster to seize on opportunities and counter issues.
Powering the programme
One of the keys to making this successful is reducing friction and optimising the speed of the systems involved. If end-users have to wait for insights or their dashboards prove laggy, then there's a risk they'll ignore the systems and revert to using their gut instinct instead. What's more, there's no point collecting data at speed or in real-time if your systems don't have the performance to match.
This is where Intel's latest Xeon Scalable processors come in. They're built to handle the most demanding, data-intensive analytics applications and extract insights in seconds rather than minutes. Their new AVX-512 instructions accelerate performance in analytics workloads, with speed improvements of between 1.4 times and 4.6 times over the previous generation in common advanced analytics applications. What's more, Intel Xeon Scalable processors are designed to work hand-in-hand with the latest Intel Optane storage technology, where enhanced performance in write-intensive tasks can result in a 2x speed improvement in SAS.
Meanwhile, Xeon Scalable and Intel Optane DC Persistent Memory enable a wider range of enterprises to make use of powerful, real-time analytics, so that they get the information they need to make the right decision at the speed today's business demands. You can think of Optane DC Persistent Memory as delivering the capacity and affordability of flash with near-DRAM levels of performance and in a standard DIMM form factor. This gives firms what they need to run real-time analytics on an in-memory database, but at a fraction of the cost this once involved.
Using analytics to make better decisions involves a combination of the right data, the right process and the right technology to power it. The data and the processes are up to you, but Intel has the technology portfolio, for the data centre, to deliver on the rest.
Discover how analytics can transform your business at intel.co.uk
Get the ITPro daily newsletter
Sign up today and you will receive a free copy of our Future Focus 2025 report - the leading guidance on AI, cybersecurity and other IT challenges as per 700+ senior executives
ITPro is a global business technology website providing the latest news, analysis, and business insight for IT decision-makers. Whether it's cyber security, cloud computing, IT infrastructure, or business strategy, we aim to equip leaders with the data they need to make informed IT investments.
For regular updates delivered to your inbox and social feeds, be sure to sign up to our daily newsletter and follow on us LinkedIn and Twitter.
-
Cleo attack victim list grows as Hertz confirms customer data stolen
News Hertz has confirmed it suffered a data breach as a result of the Cleo zero-day vulnerability in late 2024, with the car rental giant warning that customer data was stolen.
By Ross Kelly
-
Lateral moves in tech: Why leaders should support employee mobility
In-depth Encouraging staff to switch roles can have long-term benefits for skills in the tech sector
By Keri Allan
-
IDC MarketScape: Worldwide supply chain Oracle ecosystem services vendor assessment
Whitepaper In-depth assessment of IT consulting providers supporting supply chain management processes
By ITPro
-
Gartner: Data analytics teams failing to deliver benefits despite rising budgets
News Human-related challenges, such as lack of talent, were highlighted as key impediments to data strategy success
By Ross Kelly
-
Four steps to better business decisions
Whitepaper Determining where data can help your business
By ITPro
-
Boeing taps Aireon’s space data stream for improved flight safety
News ADS-B data from Aireon will further strengthen Boeing’s data and safety analytics tools
By Praharsha Anand
-
Datadog launches Observability Pipelines for enterprise data management
News The service lets businesses centrally analyze massive volumes of application metrics, logs, and traces
By Praharsha Anand
-
Building a modern strategy for analytics and machine learning success
Whitepaper Turning into business value
By ITPro
-
Telefónica Tech acquires Microsoft partner Incremental for £175m
News Glasgow-based Microsoft Dynamics partner will strengthen Telefonica Tech’s presence in the UK and Ireland
By Daniel Todd
-
Oracle analytics for dummies
Whitepaper Freedom from data overload
By ITPro