Amazon’s HR proves artificial intelligence is truly dumb
We don't know how neural networks see our world, and that's hilarious and scary
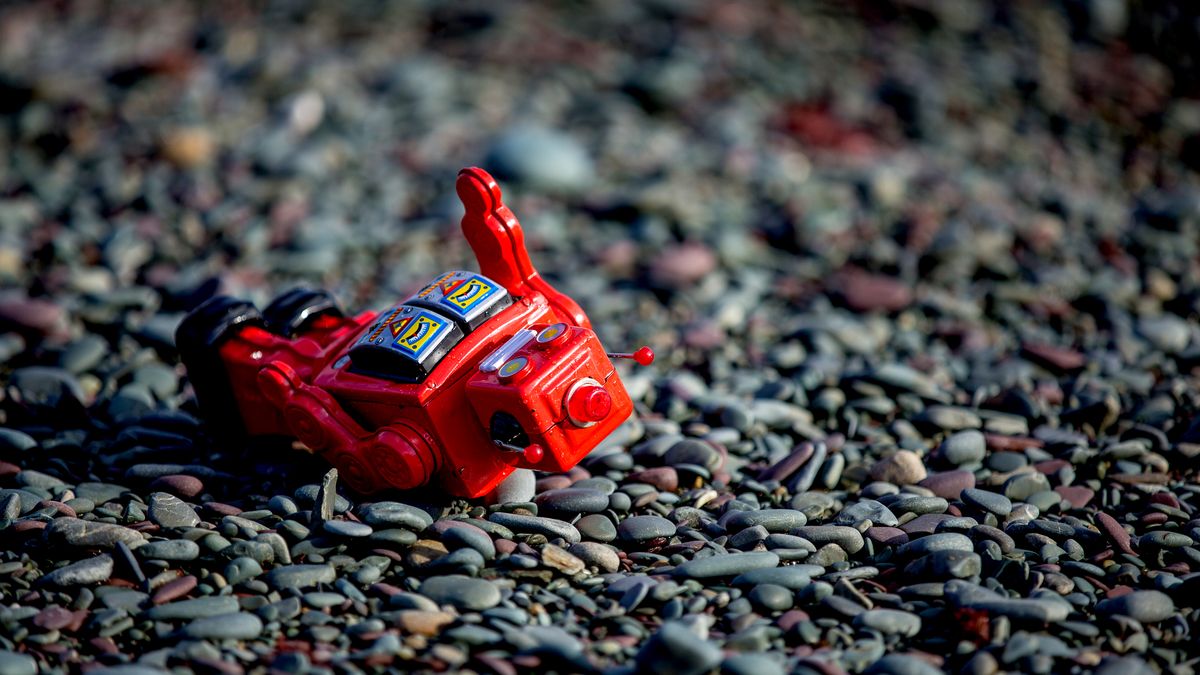
Amazon built an AI system to sift through CVs, and it turned out not to like women a fact that doesn't so much highlight algorithmic bias as it does reveal the stupidity of much of the AI that's already in use.
In 2014, Amazon started investigating ways to automate its hiring. "They literally wanted it to be an engine where I'm going to give you 100 resumes, it will spit out the top five, and we'll hire those," a source told Reuters, which uncovered the project. Within a year, it was clear the system was discounting women as candidates for technical jobs, such as software development roles. Unable to fix it, the company scrapped the entire programme.
This isn't a surprise. First, neural networks are only as good as the dataset used to train them. Suppose you wanted to train a network to spot signs of cancer in medical images. You would show it a huge database of images, telling it which ones featured cancer and which ones didn't, and it would unpick the difference between the two. It wouldn't know what cancer is or who the patients are it's merely looking for anomalies. Because that's relatively simple, it works well when trained with high-quality, physician-labelled datasets. If the training data is incomplete, limited or incorrect, then the neural network will reflect those flaws.
In other words, it's garbage in, garbage out. That's what reportedly tripped up Amazon. The HR department fed the neural network ten years of CVs of previous hires, and so naturally the system looked for staff who were like the ones already working there; all it did was replicate existing hiring practices, but more quickly.
And Amazon, like other tech companies, has a diversity problem its workforce is actually 40% female, but that falls to fewer than a quarter in managerial positions. Because the system saw that fewer women were being hired, it avoided them.
That's an oversimplification: the system has no idea what gender is, it doesn't know what a woman is, and it doesn't understand systemic workplace discrimination. It isn't sexist, it's too stupid for that. The neural network, trained on flawed data, simply noted that the word "women" such as in the phrase "women's college" or "head of the women's chess club" was found on unsuccessful CVs and not on the resumes of the people that Amazon hired, so it tossed them onto the reject pile.
Such a flaw can't be easily corrected, although Amazon reportedly tried. That's because neural networks don't actually think like we do. We assume that the AI spotted words such as "women", but men and women could have used different language or phrasing, or some other signal unseen to us. Researchers have managed to fool neural networks by adding in background noise that we humans can't see. In one case, a toy turtle looked to the machine like a rifle; in another, a stop sign was seen as a speed sign. We don't know whether it's the colour, or the shape, or something else that the network is using to identify an object. We don't see the same.
Get the ITPro. daily newsletter
Receive our latest news, industry updates, featured resources and more. Sign up today to receive our FREE report on AI cyber crime & security - newly updated for 2024.
Another lesson in what we don't know about how neural networks think comes via Janelle Shane, who offers hilarious examples of the strange way neural networks see the world via her "AI Weirdness" blog (aiweirdness.com). She trained her neural network on Sherwin-Williams paint colours, then asked it to come up with its own names. The results included "Sudden Pine", "Stanky Bean" and "Turdly". Her latest efforts include snake types ("Cancan Rattlesnake"), My Little Pony characters ("Creep Well") and college courses of the future ("Survivery").
And these are the machines we're going to use to organise and optimise our world? Don't believe the hype. Whenever someone sings the praises of neural networks, ask about the training data and how accuracy will be assessed especially if it's being used for sensitive tasks such as hiring, policing and governance. And remember that if Amazon can't get it right, there's no reason to think that a startup's neural network isn't going to spit out Turdly results, too.
Freelance journalist Nicole Kobie first started writing for ITPro in 2007, with bylines in New Scientist, Wired, PC Pro and many more.
Nicole the author of a book about the history of technology, The Long History of the Future.
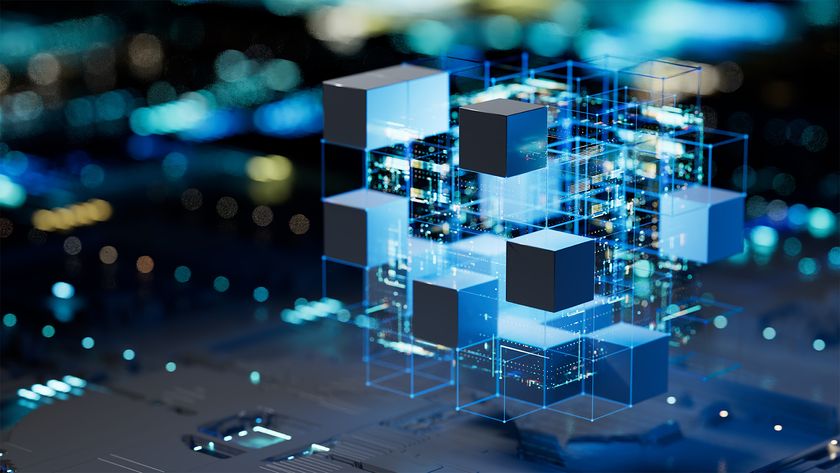
How IT decision makers can prepare for the AI Age
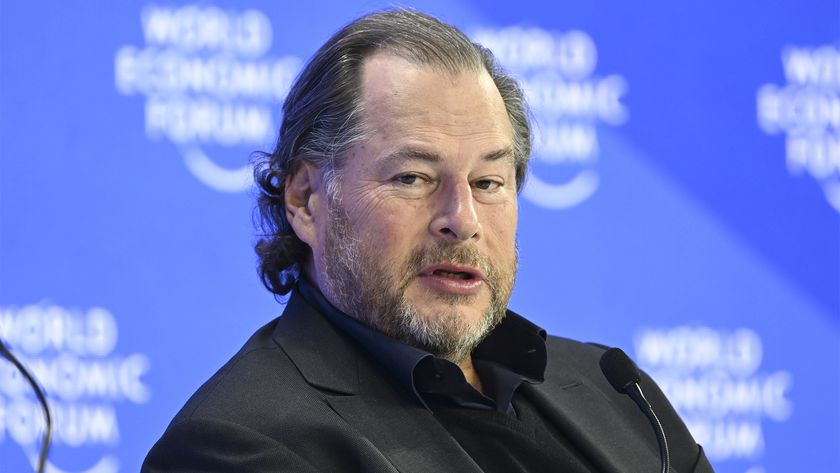
"We are really moving into a world now of managing humans and agents together": Marc Benioff thinks today’s CEOs will be the last to have a fully human workforce – and he's not the only big tech exec predicting the rise of an AI workforce
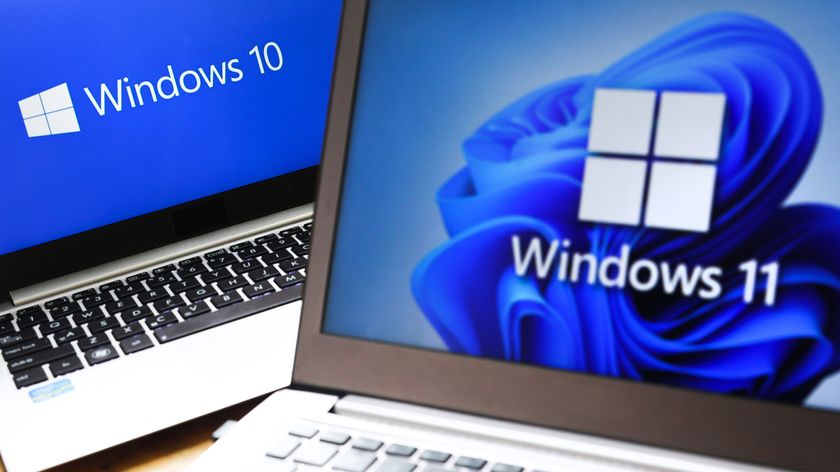
Windows 10 end of life: How to prepare for the October deadline