Nvidia and scientists teach AI to remove graininess from photos
Researchers from MIT, Aalto University and Nvidia cleans up the noise from pictures
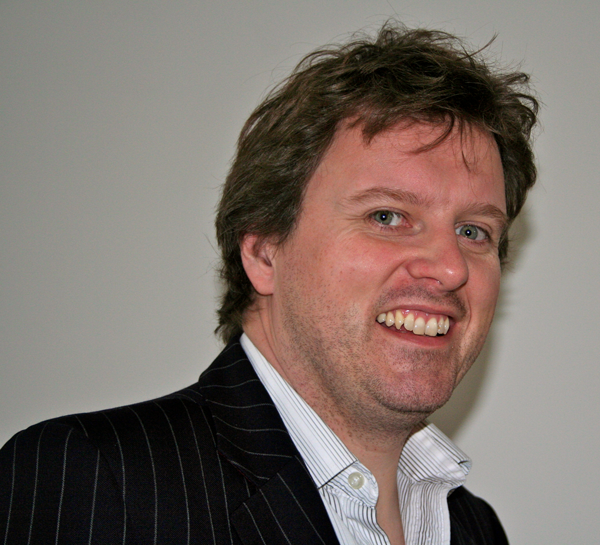
Scientists have developed an artificial intelligence system that can automatically remove noise, specks, and other distortions from pictures.
The technology, called Noise2Noise AI, was developed by researchers from Nvidia, Aalto University in Finland, and MIT. The researchers used 50,000 pictures, as well as MRI scans and computer-generated noisy images, to train the system. According to the research paper, the AI can remove enough noise to make images usable again without ever seeing a clean image.
Nvidia Tesla P100 GPUs with the cuDNN-accelerated TensorFlow deep learning framework were used to train up the system.
The technology has been trained to remove noise without needing to understand what a clean image looks like, which until now, such AI work has focused on training a neural network to restore images by showing example pairs of noisy and clean images.
"It is possible to learn to restore signals without ever observing clean ones, at performance sometimes exceeding training using clean exemplars," the researchers said.
They added that the neural network is "on par with state-of-the-art methods that make use of clean examples using precisely the same training methodology, and often without appreciable drawbacks in training time or performance".
The system was tested using three different datasets to validate the neural network.
Get the ITPro daily newsletter
Sign up today and you will receive a free copy of our Future Focus 2025 report - the leading guidance on AI, cybersecurity and other IT challenges as per 700+ senior executives
Scientists said there were several real-world situations where obtaining clean training data is difficult: low-light photography (e.g., astronomical imaging), physically-based image synthesis, and magnetic resonance imaging.
"Our proof-of-concept demonstrations point the way to significant potential benefits in these applications by removing the need for potentially strenuous collection of clean data," said the paper's authors. "Of course, there is no free lunch we cannot learn to pick up features that are not there in the input data but this applies equally to training with clean targets."
Rene Millman is a freelance writer and broadcaster who covers cybersecurity, AI, IoT, and the cloud. He also works as a contributing analyst at GigaOm and has previously worked as an analyst for Gartner covering the infrastructure market. He has made numerous television appearances to give his views and expertise on technology trends and companies that affect and shape our lives. You can follow Rene Millman on Twitter.
-
What AI models are best for vibe coding?
News Vibe coding had become the latest big trend in software development, but research shows this can yield decidedly insecure code.
By Emma Woollacott
-
Healthcare organizations are turning a blind eye to phishing attacks
News A survey reveals that most attacks go unreported, putting patient data at risk
By Emma Woollacott
-
Intel targets AI hardware dominance by 2025
News The chip giant's diverse range of CPUs, GPUs, and AI accelerators complement its commitment to an open AI ecosystem
By Rory Bathgate
-
Calls for AI models to be stored on Bitcoin gain traction
News AI model leakers are making moves to keep Meta's powerful large language model free, forever
By Rory Bathgate
-
Why is big tech racing to partner with Nvidia for AI?
Analysis The firm has cemented a place for itself in the AI economy with a wide range of partner announcements including Adobe and AWS
By Rory Bathgate
-
Baidu unveils 'Ernie' AI, but can it compete with Western AI rivals?
News Technical shortcomings failed to persuade investors, but the company's local dominance could carry it through the AI race
By Rory Bathgate
-
OpenAI announces multimodal GPT-4 promising “human-level performance”
News GPT-4 can process 24 languages better than competing LLMs can English, including GPT-3.5
By Rory Bathgate
-
ChatGPT vs chatbots: What’s the difference?
In-depth With ChatGPT making waves, businesses might question whether the technology is more sophisticated than existing chatbots and what difference it'll make to customer experience
By John Loeppky
-
Bing exceeds 100m daily users in AI-driven surge
News A third of daily users are new to the past month, with Bing Chat interactions driving large chunks of traffic for Microsoft's long-overlooked search engine
By Rory Bathgate
-
OpenAI launches ChatGPT API for businesses at competitive price
News Developers can now implement the popular AI model within their apps using a few lines of code
By Rory Bathgate