MIT researchers teach AI to spot depression
Researchers used machine learning to build a neural network to recognise the signs of depression in speech and text
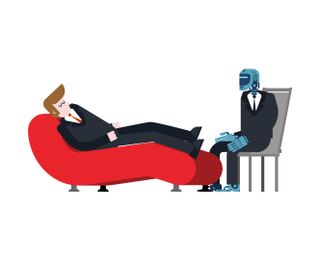
Researchers at MIT have created a neural network that can be used to spot the signs of depression in human speech.
In a paper being presented at the Interspeech Conference, the researchers detail a neural-network model that can be unleashed on raw text and audio data from interviews to discover speech patterns indicative of depression.
"The first hints we have that a person is happy, excited, sad, or has some serious cognitive condition, such as depression, is through their speech," says first author Tuka Alhanai, a researcher in the Computer Science and Artificial Intelligence Laboratory.
It is so advanced, the researchers say that given a new subject, it can accurately predict if the individual is depressed, without needing any other information about the questions and answers.
"If you want to deploy depression-detection models in a scalable way, you want to minimize the number of constraints you have on the data you're using. You want to deploy it in any regular conversation and have the model pick up, from the natural interaction, the state of the individual," said Alhanai.
It is hoped this method has the potential to be developed as a tool to detect the signs of depression in natural conversation, such as a mobile app that monitors a user's text and voice for mental distress and send alerts.
The researchers' model was trained and tested on a dataset of 142 interactions from audio, text, and video interviews of patients with mental-health issues and virtual agents controlled by humans.
Get the ITPro. daily newsletter
Receive our latest news, industry updates, featured resources and more. Sign up today to receive our FREE report on AI cyber crime & security - newly updated for 2024.
Each subject was scored in terms of depression on a scale between 0 to 27, using a personal health questionnaire. Scores between 10 to 14 were considered moderate and those between 15 to 19 were considered depressed, while all others below that threshold were considered not depressed. Out of all the subjects in the dataset, 20% were labelled as depressed.
A key insight from the research was that during experiments, the model needed much more data to predict depression from audio than it did text. With text, the model accurately detects depression using an average of seven question-answer sequences. Whereas with audio, the model needed around 30 sequences.
"That implies that the patterns in words people use that are predictive of depression happen in shorter time span in text than in audio," Alhanai added.
Bobby Hellard is ITPro's Reviews Editor and has worked on CloudPro and ChannelPro since 2018. In his time at ITPro, Bobby has covered stories for all the major technology companies, such as Apple, Microsoft, Amazon and Facebook, and regularly attends industry-leading events such as AWS Re:Invent and Google Cloud Next.
Bobby mainly covers hardware reviews, but you will also recognize him as the face of many of our video reviews of laptops and smartphones.