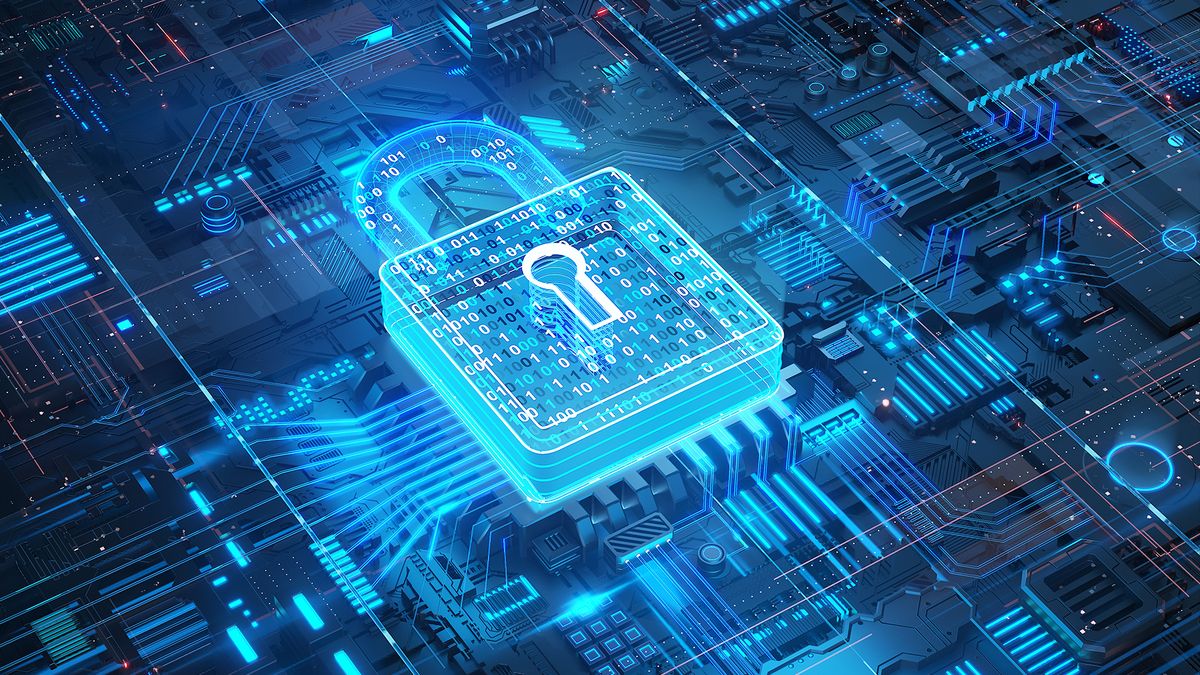
As AI moves from buzzword to business necessity, organizations face a critical challenge: protecting their data while leveraging the technology’s transformative power.
Every day, companies feed massive datasets into AI systems for everything from customer service to financial forecasting, creating new vulnerabilities that traditional security measures weren't designed to address.
The stakes are higher than ever. When AI systems process sensitive data, the risks extend beyond simple data breaches. Training data can be exposed, model outputs can reveal confidential information, and the complex nature of AI systems creates novel attack surfaces that many organizations are still learning to defend.
What begins as an effort to enhance business operations through AI can quickly become a security challenge if proper data governance isn't in place.
Whether you're just beginning to implement AI solutions or looking to strengthen existing safeguards, understanding these challenges is crucial for protecting your organization's most valuable asset – its data.
Challenges of data sovereignty with AI
Data leakage is one of the most pressing challenges when incorporating AI into organizational workflows. Whether through accidental sharing or malicious attacks, data can be exposed in ways that put the entire organization at risk. For example, in April 2023, Samsung employees put some of the company’s source code into ChatGPT, thus unwittingly making sensitive IP available to anyone. Such incidents underscore the critical need for comprehensive governance to protect data integrity and minimize exposure risks.
The inherent complexity of data governance grows as AI is integrated into business processes, which produces yet more challenges. Organizations not only face traditional data privacy and security issues, but also have to navigate new challenges introduced by the nature of AI itself. AI models often require extensive datasets from multiple sources, which can lead to difficulties in maintaining data integrity and complying with regional regulations on data privacy and sovereignty.
Additionally, the intermixing of data from different regions further complicates compliance, creating a need for meticulous oversight and rigorous data handling procedures.
Examples of data leakage in AI workflows
Data leakage is a major risk when using AI, and there are numerous scenarios where organizations could face significant consequences. For instance, consider a financial institution that uses customer data to train an AI model intended to enhance personalized marketing.
Without adequate data masking and oversight, there could be accidental exposure of customer financial details, causing reputational damage and potential regulatory scrutiny. This scenario underscores the importance of implementing stringent data masking and anonymization techniques when using sensitive information for AI training.
Another scenario could involve a healthcare provider partnering with an AI vendor to develop predictive analytics for patient outcomes. During the collaboration, if data sharing practices fail to comply with regional privacy laws, it could lead to regulatory fines and public backlash. The lesson here is clear: organizations must ensure compliance with data sovereignty regulations before sharing any data externally, especially when collaborating with third-party AI developers.
These examples demonstrate that effective data governance — incorporating practices like data masking, stringent access controls, and strict adherence to localization requirements — is crucial to minimizing risk and ensuring compliance when working with AI.
The role of data governance in AI governance
Effective AI governance must start with data governance, as AI models are inherently dependent on data to function. Without proper governance of the data feeding these models, any AI governance effort becomes flawed.
Data governance forms the foundation of AI governance by ensuring data integrity, protection, and appropriate access control. The link between data governance and AI governance is inseparable — without trustworthy data, AI models are prone to errors, bias, and potential misuse. Protecting data and controlling who can access it helps mitigate the risks of data breaches and model corruption, leading to a more secure AI environment.
A well-governed dataset will reduce the chances of biased outcomes by ensuring data quality and consistency. Strong data protection measures, such as encryption and access control, also prevent unauthorized individuals from altering data, which could otherwise compromise the AI model’s outputs. In essence, data integrity, protection, and access control are the building blocks for robust AI governance.
Strategies for effective data governance
To support effective AI governance, organizations must focus on several key strategies:
- Maintaining data integrity: Organizations should implement encryption, employ a Zero Trust model, and conduct regular data audits to maintain data integrity throughout the AI lifecycle. Data audits help track changes and identify potential vulnerabilities in the data handling process.
- Preventing data exfiltration: Preventing data loss is essential to keep sensitive information within the organization and prevent it from being used for unauthorized AI models. Data Loss Prevention (DLP) solutions, Cloud Access Security Brokers (CASB), and AI firewalls play a crucial role in mitigating data exfiltration risks.
- Controlling access and adhering to data localization rules: Ensuring proper access control is fundamental for safeguarding sensitive information used in AI models. Zero Trust solutions can help organizations manage internal access, ensuring that only authorized personnel have access to specific data. Adhering to data localization requirements is also critical, particularly when dealing with regional compliance standards such as GDPR. Data localization controls can ensure that data remains within the appropriate jurisdiction, helping organizations comply with these regulations.
Moving forward with strong data governance
Strengthening data governance is a critical step that organizations must take now to ensure the success of their AI initiatives. The evolving nature of AI means that the data underpinning these models needs to be handled with meticulous care to prevent exposure and minimize risks.
Connectivity cloud solutions offer a powerful way to support data governance efforts. By providing a unified platform of cloud-native services, these solutions can help organizations regain control over data, secure it throughout its lifecycle, and adhere to compliance requirements without the complexity of managing disparate tools.
The long-term benefits of enhanced data governance are multifaceted. Improved governance not only helps protect data integrity and privacy but also boosts the reliability and scalability of AI models. Organizations that invest in strong data governance today will be better positioned to derive value from AI, with fewer risks and greater compliance.
Get the ITPro. daily newsletter
Receive our latest news, industry updates, featured resources and more. Sign up today to receive our FREE report on AI cyber crime & security - newly updated for 2024.
ITPro is a global business technology website providing the latest news, analysis, and business insight for IT decision-makers. Whether it's cyber security, cloud computing, IT infrastructure, or business strategy, we aim to equip leaders with the data they need to make informed IT investments.
For regular updates delivered to your inbox and social feeds, be sure to sign up to our daily newsletter and follow on us LinkedIn and Twitter.