Hackers are deliberately "poisoning" AI systems to make them malfunction, and there's no way to defend against it
NIST has warned there is no surefire way to protect against AI model "poisoning" attacks, which are growing in both intensity and scale
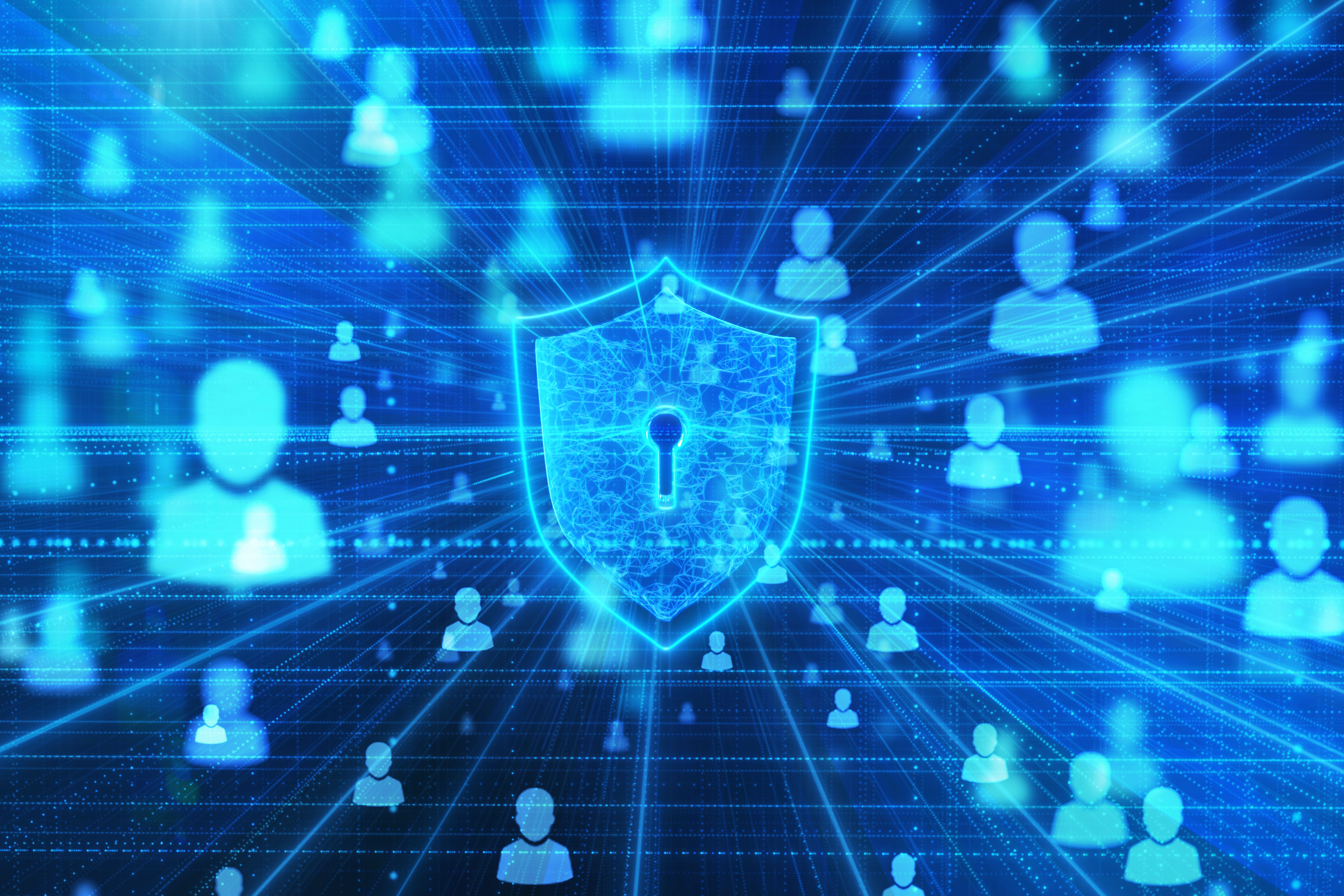
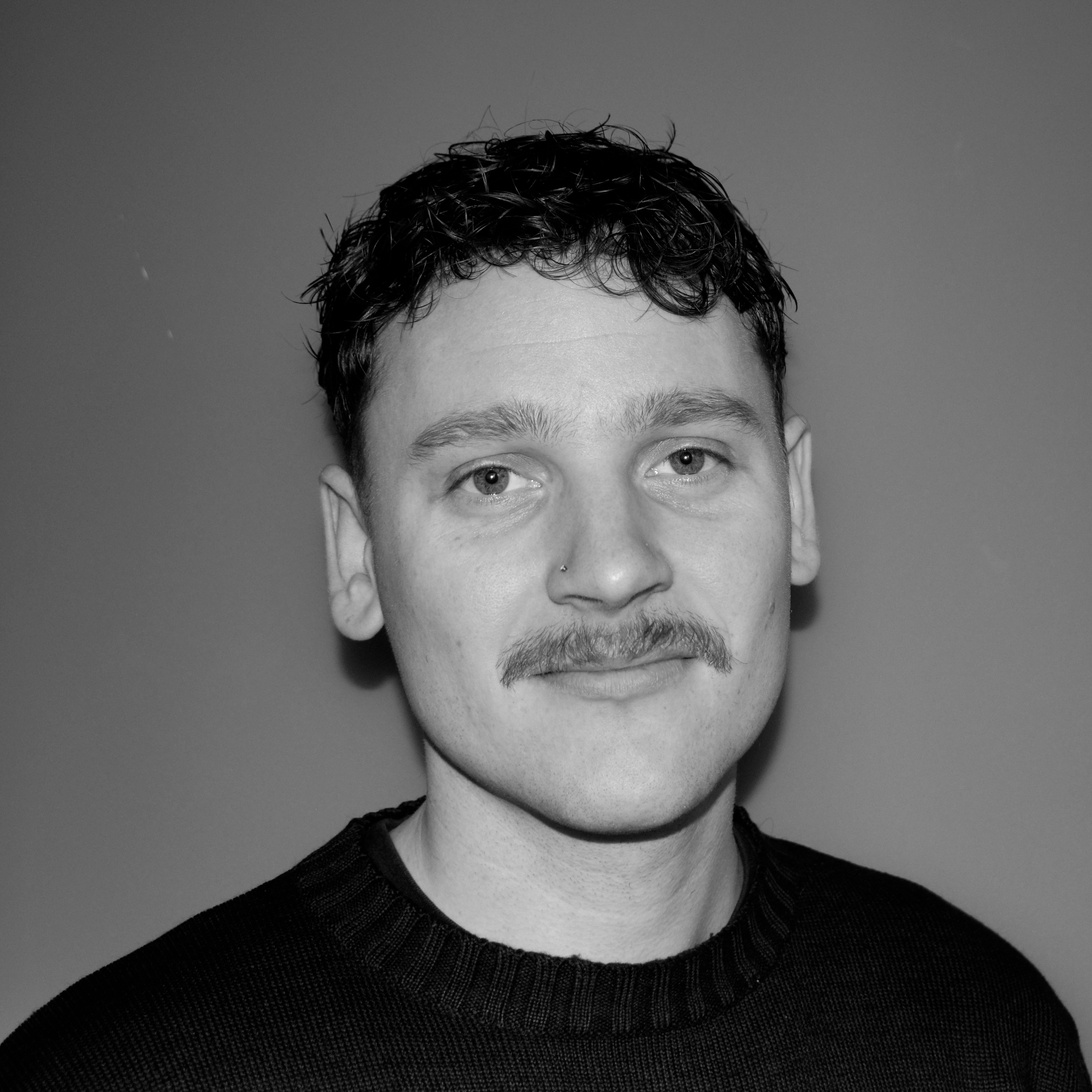
Threat actors are exploiting malfunctioning AI systems caused by “untrustworthy data”, a new advisory from the National Institute of Standards and Technology (NIST) warns.
The new NIST report on trustworthy and responsible AI reveals how hackers can intentionally ‘poison’ AI systems and cause them to malfunction, which they can then exploit.
Worryingly, the report also notes developers have no foolproof way to guard against attacks of this nature.
One of the report’s authors, NIST computer scientist Apostol Vassilev, said current mitigation strategies used to combat attacks of this nature cannot guarantee protection.
“We also describe current mitigation strategies reported in the literature, but these available defenses currently lack robust assurances that they fully mitigate the risks,” he said. “We are encouraging the community to come up with better defenses.”
Adding to the problems facing organizations deploying AI, the attack methods outlined in the NIST’s report require little prior knowledge of the system itself and are not limited to the most sophisticated threat actors.
Co-author, professor Alina Oprea of Northeastern University, noted, “[m]]ost of these attacks are fairly easy to mount and require minimum knowledge of the AI system and limited adversarial capabilities.”
Get the ITPro daily newsletter
Sign up today and you will receive a free copy of our Future Focus 2025 report - the leading guidance on AI, cybersecurity and other IT challenges as per 700+ senior executives
Four major attack types used to target vulnerable AI systems
The NIST report outlines the four primary types of attacks used to compromise AI technologies: poisoning, evasion, privacy, and abuse attacks.
Poisoning attacks require hackers to access the model during the training phase, using corrupted data to fundamentally alter the system’s inferences. By feeding the AI system unvetted data the attackers are able to influence how it will behave once it is deployed.
For example, a chatbot could be made to generate offensive or threatening responses to prompts by injecting malicious content into the model while it is being trained.
Similar to poisoning attacks, abuse attacks also where data used to train the data is used to create vulnerabilities in the system. Abuse differs in that, instead of inserting malicious content used to produce undesired behaviors from the AI, abuse attacks use incorrect information that comes from a purportedly legitimate source in order to compromise the system.
RELATED RESOURCE
Discover the four steps CIOs can follow to optimize spend
DOWNLOAD NOW
Evasion attacks take place after the deployment of an AI system and involve threat actors using subtle alterations in inputs to try and skew the model’s intended function.
One example provided in the report included using small changes in traffic signs to cause an autonomous vehicle to misinterpret them and respond in potentially dangerous, unprescribed ways.
Privacy attacks also occur during the deployment phase of an AI systems lifecycle. A privacy attack involves threat actors interacting with the AI system to gain information on the AI which they can then use to pinpoint weaknesses they can exploit.
Chatbots provide a clear example of this type of attack vector, where attackers can ask the AI legitimate, seemingly harmless questions and use the answers to improve their understanding of the data used to train the model.
As with poisoning and abuse attacks, being able to influence the data that models are trained on ultimately leads to being able to influence the AI system, and so privacy attacks also build on this concept by trying to get the AI itself to give away vital information that could be used to compromise the system.
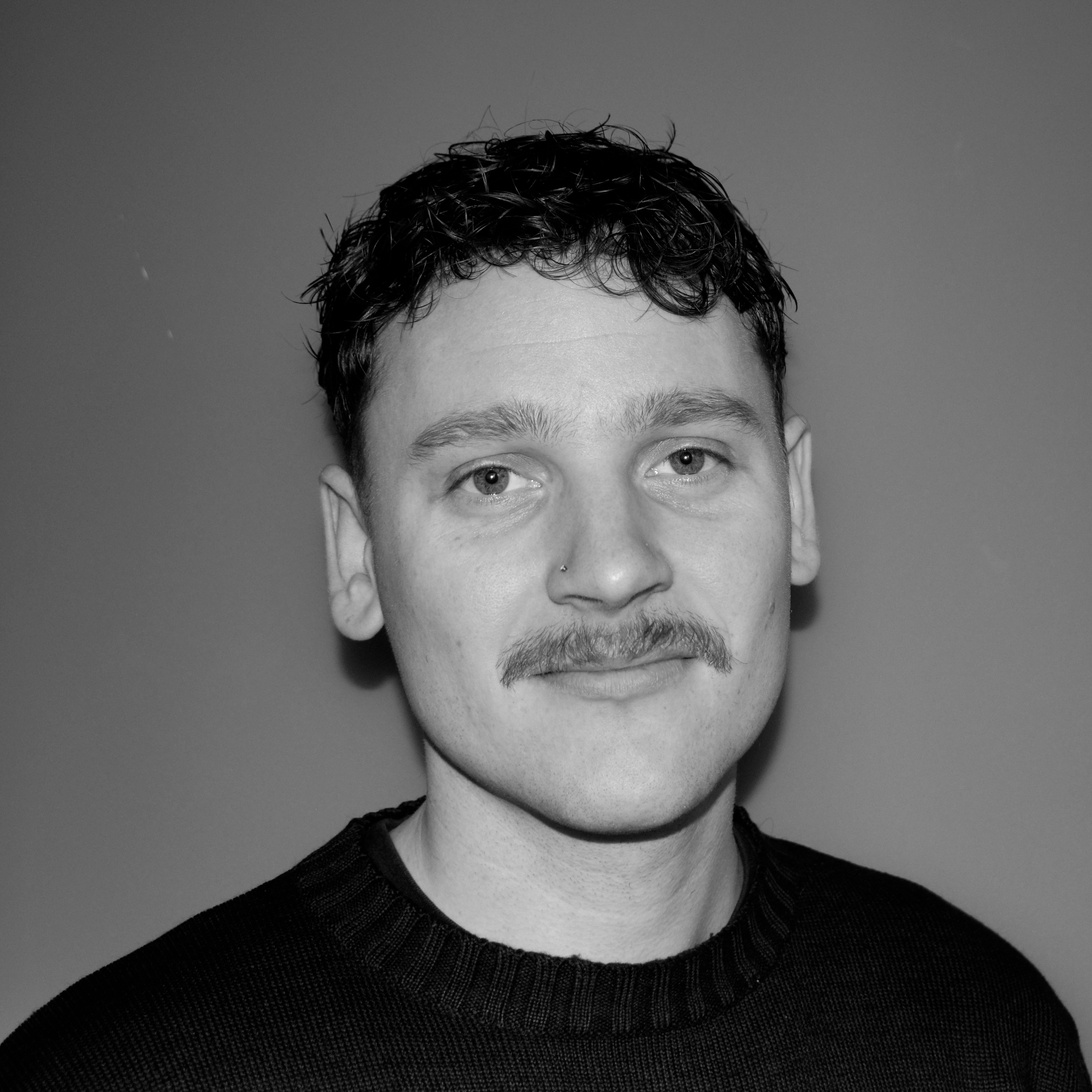
Solomon Klappholz is a former staff writer for ITPro and ChannelPro. He has experience writing about the technologies that facilitate industrial manufacturing, which led to him developing a particular interest in cybersecurity, IT regulation, industrial infrastructure applications, and machine learning.
-
Why are many men in tech blind to the gender divide?
In-depth From bias to better recognition, male allies in tech must challenge the status quo to advance gender equality
By Keri Allan
-
BenQ PD3226G monitor review
Reviews This 32-inch monitor aims to provide the best of all possible worlds – 4K resolution, 144Hz refresh rate and pro-class color accuracy – and it mostly succeeds
By Sasha Muller