Proofpoint touts the benefits of having smaller models in its cybersecurity tools
The firm suggests that smaller models are more secure to use and more efficient to run
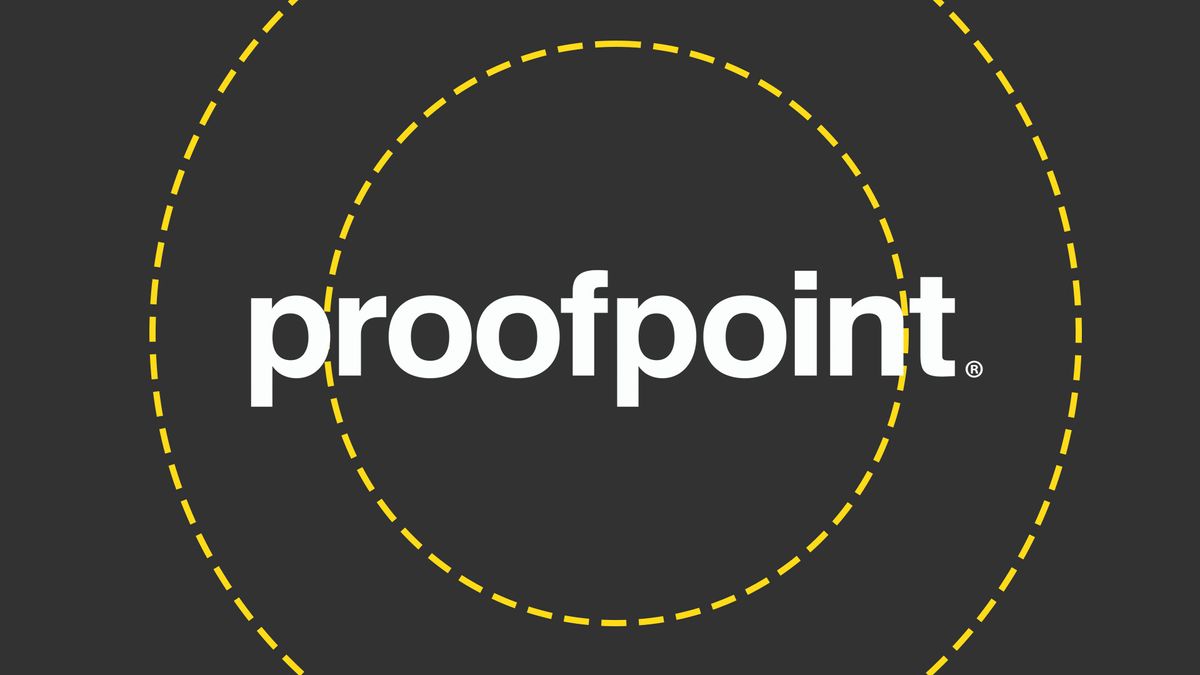
Proofpoint is focused on having smaller, more efficient AI models to power the company’s cybersecurity tools, according to execs at Proofpoint Protect London 2024.
Daniel Rapp, VP of AI at Proofpoint, described the firm’s concerns on stage for the opening keynote, saying that one of the challenges it is facing is how to reduce the size of its models for greater efficiency in certain use cases.
“If I were perhaps writing a dissertation on English literature, I might want a model to understand the whole works of Shakespeare - but threat actors really aren't quoting Hamlet,” Rapp said.
“So what I need is a model that is detecting deceptive language rather than detecting whether or not an email is delivered in blank verse,” Rapp added.
Essentially, Rapp wants to make Proofpoint’s models “more computationally effective,” and he outlined some key techniques the firm can use to do this, such as pruning the model size. This is done via a process of quantization or distillation, Rapp explained. The latter of which Proofpoint has applied to Nexus, the firm’s AI platform. Distillation involves training a smaller model to “mimic” some of a larger model’s key characteristics for certain use cases.
In a separate media roundtable, Ryan Kalember, EVP of cybersecurity strategy at Proofpoint, went into more detail on the advantages of reduced-size models. One such advantage was protection from abuse.
“If you can prompt the model with more things, that introduces risk,” Kalember said in response to a question from ITPro. “The vast majority of attacks that we have seen against language models involve having to be able to interface with them directly.”
Get the ITPro. daily newsletter
Receive our latest news, industry updates, featured resources and more. Sign up today to receive our FREE report on AI cyber crime & security - newly updated for 2024.
“So when we look at smaller models that are doing discrete things, they're a lot less risky, just for that reason,” he added.
Kalember said that, if nothing other than Proofpoint’s internal APIs interfacing with the models, it’s less likely that the models could be poisoned and it’s less likely that there could be any sort of model abuse.
Smaller models are all the rage
Small language models (SLMs) are becoming increasingly popular as enterprises look to cut down on the costs associated with training or deploying large language models (LLMs).
OpenAI’s release of GPT-4o mini put SLMs in focus recently, and its price point of 5 cents per million input tokens and 60 cents per million output tokens makes this lightweight model 60% cheaper than GPT-3.5 Turbo.
At the time, however, experts told ITPro there was a “hidden fallacy” in SLMs - founder and CEO of Articul8, Arun Subramaniyan, said that enterprises would eventually find them insufficient to “get to production.”
George Fitzmaurice is a staff writer at ITPro, ChannelPro, and CloudPro, with a particular interest in AI regulation, data legislation, and market development. After graduating from the University of Oxford with a degree in English Language and Literature, he undertook an internship at the New Statesman before starting at ITPro. Outside of the office, George is both an aspiring musician and an avid reader.