Machine learning vs AI vs NLP: What are the differences?
AI, machine learning, and natural language processing are terms often used interchangeably, but they are drastically different technologies
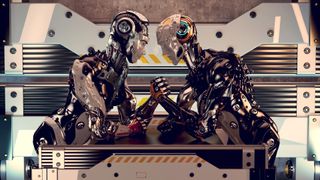
The question of machine learning (ML) vs AI has been a common one ever since OpenAI’s release of its generative AI platform ChatGPT in November 2022. The preceding COVID-19 pandemic drove businesses to accelerate their digital transformation, moving at a pace far quicker than anyone thought feasible, kicking off a technological arms race as firms battled to stay ahead of the curve with bigger and more ambitious projects.
Magic quadrant for enterprise conversational AI platforms
An evaluation of the conversational AI platform (chatbot) market
AI and ML reflect the latest digital inflection point that has caught the eye of technologists and businesses alike, intrigued by the various opportunities they present. Ever since Sam Altman announced the general availability of ChatGPT, businesses throughout the tech industry have rushed to take advantage of the hype around generative AI and get their own AI/ML products out to market.
Despite the two technologies occupying an increasing area of column inches over recent years, there remains a great deal of confusion around what they actually are, and how they differ from each other. This confusion is only exacerbated by the explosion of interest in natural language processing (NLP) tools after the world saw how ChatGPT could mimic human conversation so convincingly. So what exactly are the differences between AI, ML, and NLP?
Most likely you will have heard AI and ML mentioned in the same breath. Neither AI nor ML can function without the other, but there are a number of clear distinctions between the two.
AI has myriad uses, often to do with automation and anomaly detection. Some common examples in business would be fraud protection, customer service, and statistical analysis for pricing models.
While these examples of the technology ave been largely 'behind the scenes', more human-friendly AI has emerged in recent years, culminating with generative AI.
If you haven’t come across generative AI tools like ChatGPT or Google Gemini, then perhaps you will be familiar with some slightly older applications of this technology such as voice assistants like Amazon’s Alexa or Apple’s Siri.
Machine learning, on the other hand, has a slightly narrower scope, often thought of as the using statistical algorithms to build knowledge from a specific dataset, imitating the way humans learn and then extrapolating this knowledge and applying it to new datasets. ML can be broken down into two subcategories: deep learning using neural networks focusing trained on a dataset, or reinforcement learning which learns dynamically with a more trial-and-error style approach adjusting to feedback as it goes.
As for NLP, this is another separate branch of AI that refers to the ability of a computer program to understand spoken and written human language, which is the “natural language” part of NLP. This helps computers to understand speech in the same way that people do, no matter if it’s spoken or written. This makes communication between humans and computers easier and has a range of use cases.
What's the difference between ML and AI?
The origins of AI as a concept go back a long way, often far deeper in time than most people think. Humans have dreamed up machines that could behave and think just like themselves for millennia and some of the earliest computers were often considered a form of artificial intelligence due to the logical way in which they processed information.
In its current manifestation, however, the idea of AI can trace its history to British computer scientist and World War II codebreaker Alan Turing. He proposed a test, which he called the imitation game but is more commonly now known as the Turing Test, where one individual converses with two others, one of which is a machine, through a text-only channel. If the interrogator is unable to tell the difference between the machine and the person, the machine is considered to have "passed" the test.
This basic concept is referred to as 'general AI' and is generally considered to be something that researchers have yet to fully achieve.
However, 'narrow' or 'applied' AI has been far more successful at creating working models. Rather than attempt to create a machine that can do everything, this field attempts to create a system that can perform a single task as well as, if not better than, a human.
It's within this narrow AI discipline that the idea of machine learning first emerged, as early as the middle of the twentieth century. First defined by AI pioneer Arthur Samuel in a 1959 academic paper, ML represents "the ability to learn without being explicitly programmed".
What is machine learning used for?
Interest in ML has waxed and waned over the years, but with data becoming an increasingly important part of business strategy, it's fallen back into favour as organizations seek ways to analyze and make use of the vast quantities of information they collect on an almost constant basis.
When this data is put into a machine learning program, the software not only analyzes it but learns something new with each new dataset, becoming a growing source of intelligence. This means the insights that can be learnt from data sources become more advanced and more informative, helping companies develop their business in line with customer expectations.
One application of ML is in a recommendation engine, like Facebook's newsfeed algorithm, or Amazon's product recommendation feature. ML can analyze how many people are liking, commenting on or sharing posts or what other people are buying that have similar interests. It will then show the post to others the system thinks will like it.
Magic quadrant for enterprise conversational AI platforms
An evaluation of the conversational AI platform (chatbot) market
ML is also particularly useful for image recognition, using humans to identify what's in a picture as a kind of programming and then using this to autonomously identify what's in a picture. For example, machine learning can identify the distribution of the pixels used in a picture, working out what the subject is.
Enterprises are now turning to ML to drive predictive analytics, as big data analysis becomes increasingly widespread. The association with statistics, data mining and predictive analysis have become dominant enough for some to argue that machine learning is a separate field from AI.
The reason for this is that AI technology, such as natural language processing or automated reasoning, can be done without having the capability for machine learning. It is not always necessary for ML systems to have other features of AI.
What is AI used for?
There are hundreds of use cases for AI, and more are becoming apparent as companies adopt artificial intelligence to tackle business challenges.
One of the most common uses of AI is for automation in cyber security. For example, AI algorithms can be programmed to detect threats that may be difficult for a human to spot, such as subtle changes in user behavior or an unexplained increase in the amount of data being transferred to and from a particular node (such as a computer or sensor). In the home, assistants like Google Home or Alexa can help automate lighting, heating and interactions with businesses through chatbots.
There are well-founded fears that AI will replace human job roles, such as data input, at a faster rate than the job market will be able to adapt to. Author and venture capitalist Kai-Fu Lee, who has worked at both Apple and Google and earned a PhD from Carnegie Mellon for the development of an advanced speech recognition AI, warned in 2019 that "many jobs that seem a little bit complex, a chef, a waiter, a lot of things, will become automated."
"We will have automated stores, automated restaurants and all together, in 15 years, that's going to displace about 40% of jobs in the world."
What is NLP used for?
NLP has a variety of use cases, with a notable one being speech synthesis. This is where NLP technology is used to replicate the human voice and apply it to hardware and software. You will have encountered a form of NLP when engaging with a digital assistant, whether that be in Alexa or Siri, which analyze the spoken word in order to process an action, and then respond with an appropriate human-like answer. However, NLP is also particularly useful when it comes to screen reading technology, or other similar accessibility features.
Translation is a large area of focus for NLP. Where we at one time relied on a search engine to translate words, the technology has evolved to the extent that we now have access to mobile apps capable of live translation. These apps can take the spoken word, analyze and interpret what has been said, and then convert that into a different language, before relaying that audibly to the user. This allows people to have constructive conversations on the fly, albeit slightly stilted by the technology.
NLP is also used in natural language generation, which uses algorithms to analyse unstructured data and produce content from that data. It’s used by language models like GPT3, which can analyze a database of different texts and then generate legible articles in a similar style.
Other real-world applications of NLP include proofreading and spell-check features in document creation tools like Microsoft Word, keyword analysis in talent recruitment, stock forecasting, and more.
AI vs ML
To make matters more confusing when it comes to naming and identifying these terms, there are a number of other terms thrown into the hat. These include artificial neural networks, for instance, which process information in a way that mimics neurons and synapses in the human mind. This technology can be used for machine learning; although not all neural networks are AI or ML, and not all ML programmes use underlying neural networks.
As this is a developing field, terms are popping in and out of existence all the time and the barriers between the different areas of AI are still quite permeable. As the technology becomes more widespread and more mature, these definitions will likely also become more concrete and well known. On the other hand, if we develop generalized AI, all these definitions may suddenly cease to be relevant.
This article was first published on 28/10/2019, and has since been updated
Get the ITPro. daily newsletter
Receive our latest news, industry updates, featured resources and more. Sign up today to receive our FREE report on AI cyber crime & security - newly updated for 2024.
Jane McCallion is ITPro's Managing Editor, specializing in data centers and enterprise IT infrastructure. Before becoming Managing Editor, she held the role of Deputy Editor and, prior to that, Features Editor, managing a pool of freelance and internal writers, while continuing to specialize in enterprise IT infrastructure, and business strategy.
Prior to joining ITPro, Jane was a freelance business journalist writing as both Jane McCallion and Jane Bordenave for titles such as European CEO, World Finance, and Business Excellence Magazine.