What is Tiny AI?
Listed as one of MIT Technology Review’s 10 Breakthrough Technologies of 2020, this version of AI is set to make a real difference in solving important problems. But what exactly is it and what will its impact be?
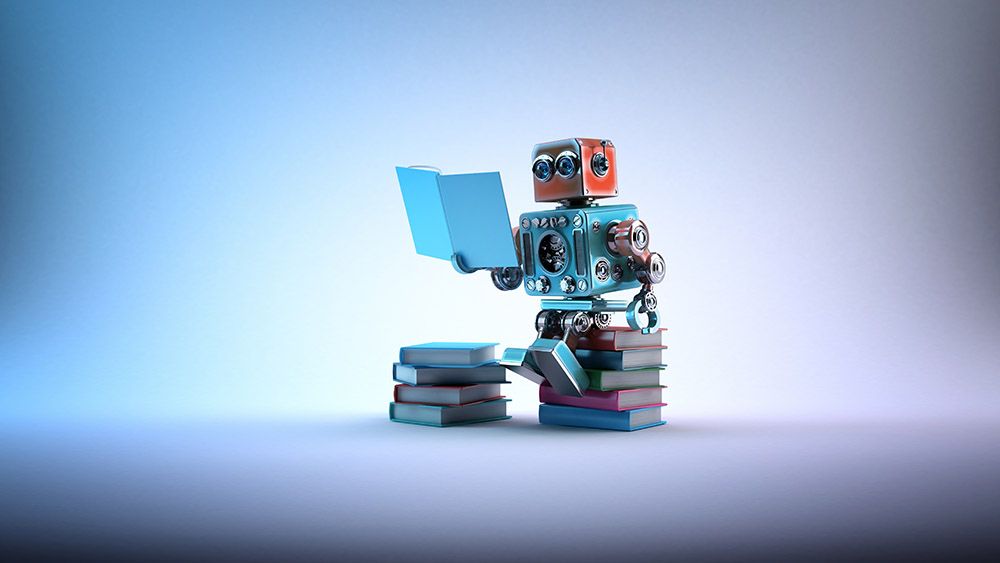
Tiny AI, also known as Tiny ML in reference to machine learning (ML), is currently a big focus for AI researchers. The aim is to reduce the size of artificial intelligence (AI) algorithms; especially those that use large quantities of data and computational power, for example natural language processing (NLP) models like Google’s BERT. According to the MIT Technology Review, the larger version of BERT has 340 million data parameters and training it just once costs enough electricity to power a US household for 50 days.
“Over the past few years there’s been an arms race of sorts in AI and an effect of this competition is that we’ve seen some ML models become enormous in the race to achieve high performance,” says Nick McQuire, vice president of enterprise research at CCS Insight. “Microsoft, for instance, recently introduced the Turing Natural Language Generation model, the largest one ever published at 17 billion parameters.”
Why do we need Tiny AI?
Training sophisticated AI takes a huge amount of energy. Sumant Kumar, director of digital transformation at consultancy firm CGI UK, notes that the carbon footprint of training a single AI is as much as 284 tonnes of carbon dioxide equivalent (CO2e) – five times as much as the lifetime emissions of an average car. As AI adoption grows it’s become clear that the technology needs to become greener, which is one of the factors pushing Tiny AI forward.
Another factor is the need to run inference and sophisticated models on resource-limited devices at the edge, for use cases like robotics, automated video security and anomaly detection in manufacturing. “To get increasing intelligence out of the data centre and into better performing consumer electronics, cars and medical devices, AI needs to run on much smaller microprocessors, often powered by batteries,” says Tim Ensor, director of AI at consultancy business Cambridge Consultants.
“Tiny AI involves building algorithms into hardware at the periphery of a network, such as the sensors themselves,” explains Andrew White, a UK and European patent attorney at IP firm Mathys & Squire. “The idea is that they can be integrated into hardware to perform data analytics at low power, avoiding the need to send data back to the cloud for processing.” This improves latency as well as power consumption and enables Tiny AI to run on devices like our mobile phones, increasing their functionality but also improving our privacy as the data stays on the device.
What’s happening in this field?
Alongside academics, tech giants including Google, IBM, Amazon and Apple are also undertaking research in this field.
“We’ve seen commercial moves in this arena, notably by the cloud providers, the highest profile of which was AWS’ launch of Sagemaker Neo last year, which is one of the leaders in this space for developers,” says McQuire.
Get the ITPro. daily newsletter
Receive our latest news, industry updates, featured resources and more. Sign up today to receive our FREE report on AI cyber crime & security - newly updated for 2024.
We’re also seeing collaborations between the two sectors. For example, TinyBERT, which is 7.5 times smaller and 9.4 times faster on inference than the original BERT, was developed by Huazhong University of Science and Technology and Huawei Noah’s Ark Lab.
The main benefit of reducing the size of machine learning is the reduction in computational power, which lowers cloud and power costs. “We’ve seen customers that for every dollar they spend on AI, spend $10-15 more on cloud computing to support the application,” notes McQuire.
The IT Pro Podcast: Can AI ever be ethical?
As AI grows in sophistication, how can we make sure it’s being developed responsibly?
But there are other benefits. The fact that Tiny AI leverages local computer resources rather than the cloud is a big benefit for highly regulated and privacy conscious industries, such as banking and healthcare, for example.
The challenges ahead
Research into Tiny AI is critical in enabling AI to realise its full potential. The challenge for both researchers and technology firms is managing the trade-off between reducing the size of a model, through distillation techniques for instance, and maintaining accuracy and high performance for inference.
As Tiny AI is closer to the human experience, the accuracy needs to be high. “There’s no room for error with things like autonomous vehicles,” notes Kumar. “It’s also vital to make Tiny AI algorithms at the edge secure, transparent and ethical, as they’ll be deployed in real-life environments.”
Tiny AI could fundamentally alter the way we interact with many devices and will be necessary to create the next wave of context-aware consumer devices. It looks set to improve a myriad services and technologies including, but not limited to, voice assistants, autocorrect, image processing in cameras, autonomous driving, precision farming, connected healthcare, Industry 4.0 and intelligent logistics. It will also make many new applications possible.
Kumar believes that Tiny AI will be essential in unlocking the business value of AI in industries such as manufacturing, oil and gas, healthcare and logistics, while McQuire expects it to change how we work.
“Many of the devices we use for work supporting Tiny AI will get much smarter, especially in the areas of security, video and voice understanding,” he says. “Whilst office work has been fundamentally changed forever as a result of COVID-19, when people finally are able to go back into face-to-face meetings in offices, we will likely see Tiny AI and IoT converge in smart offices and facilities management applications.”
When will Tiny AI become commonplace?
While this is very much an emerging area, Tiny AI represents an expected evolution of the field of AI, says Ensor. However, it’s highly research driven at the moment and there are few companies leveraging Tiny AI in production today.
Issues like governance, management tools and security still need to be hashed out, but “in coming years we will see some experimental deployments in real-life starting with low risk applications, and implementations in more critical use cases will follow as technology matures,” Kumar concludes
Keri Allan is a freelancer with 20 years of experience writing about technology and has written for publications including the Guardian, the Sunday Times, CIO, E&T and Arabian Computer News. She specialises in areas including the cloud, IoT, AI, machine learning and digital transformation.