AI hallucinations: What are they?
Believing everything generative AI tells you is not advised. If it lacks the ability to fact check itself before answering, its responses can be wildly inaccurate, something know as a ‘hallucination’
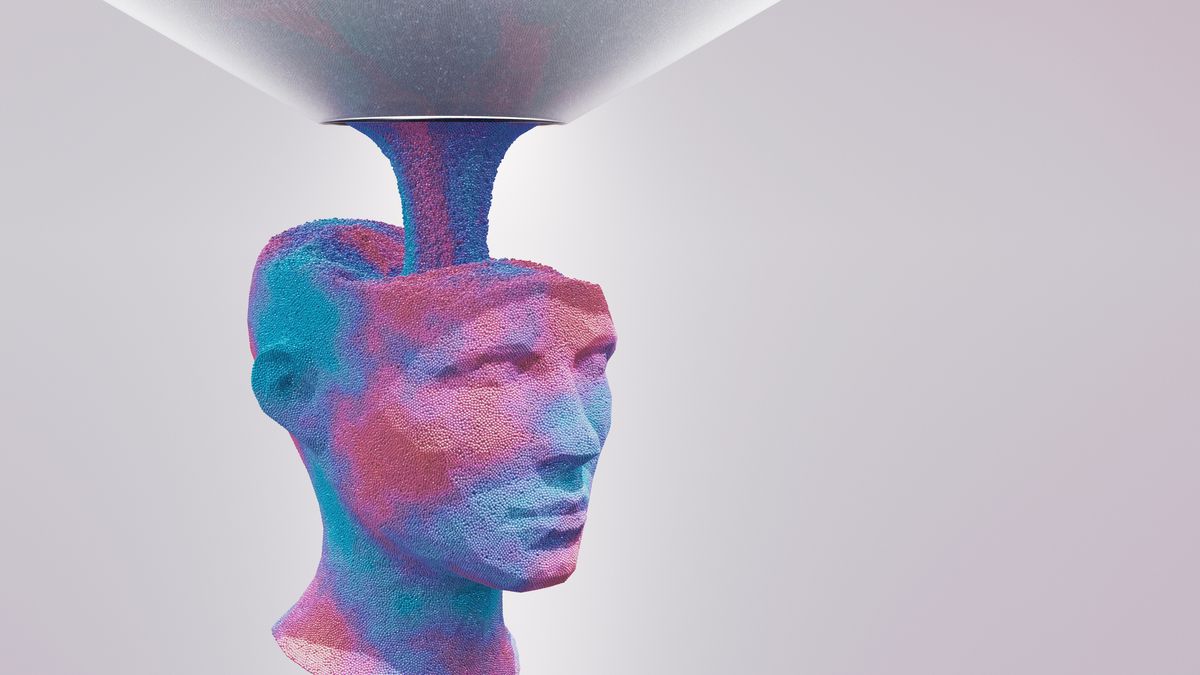
The surge in the use of AI tools globally is changing how people interact with information for both their personal and working lives. But despite its popularity, AI continues to have limitations – not least delivering inaccurate or incorrect answers to user queries.
For users, this can be difficult to navigate. Responses from large language models (LLMs) – of which Open AI’s ChatGPT is just one – can often appear to be confident and believable. However, if they are wrong in full or in part, there is a huge risk if the response is used as the basis for making critical decisions. These ill-informed answers are more commonly known as “hallucinations”.
Eleanor Lightbody, CEO at AI legal tool firm Luminance, says: “At a high level, hallucinations occur when an AI system provides factually incorrect or false information. We see them most commonly in generative AI systems like general-purpose chatbots that have ingested vast amounts of data from across the entire internet. The goal of generative AI in these generalist systems is to always provide an answer, even if that means plausible sounding but incorrect output.”
Within highly regulated industries, hallucinations can be massively problematic and potentially costly for companies and enterprises.
“Sometimes algorithms produce outputs that are not based on training data and do not follow any identifiable pattern. In other words, it hallucinates the response,” explains Simon Bennett, global CTO at Rackspace Technology.
“In a new study published in Nature, researchers found LLMs give different answers each time they are asked a question, even if the wording is identical, known as ‘confabulating’. This means it can be difficult to tell when they know an answer versus when they are simply making something up.”
Bennett adds: “AI hallucinations can have huge consequences, including contributing to the spread of misinformation. For instance, if hallucinating news bots respond to queries about a developing emergency with information that hasn’t been fact-checked, it can quickly spread falsehoods.”
Get the ITPro. daily newsletter
Receive our latest news, industry updates, featured resources and more. Sign up today to receive our FREE report on AI cyber crime & security - newly updated for 2024.
AI hallucinations: Building in biases
One cause of such hallucinations can be from biases embedded in AI models when they were trained, with Bennett describing how this may mean it will “hallucinate patterns that reflect these biases”.
“Just as racial bias has proven difficult to eliminate in the real world, eliminating bias in AI isn’t an easy task,” he adds. “This is because LLMs often rely on historical data, and this can reinforce existing patterns of inappropriate profiling and disproportionate targeting.”
According to a recent Global AI Report from Rackspace Technology, nearly 40% of IT decision-makers have expressed concern about their organizations lacking adequate safeguards to ensure responsible AI use.
Bennett warns AI hallucinations in LLMs are a “growing cyber security concern”, pointing to how bad actors can manipulate the output of an AI model by subtly tweaking the input data.
For example, in image recognition an attack might involve editing an image, causing the AI to misclassify it. Strengthening validation processes and enhanced threat detection, including secure IT protocols, is one countermeasure.
“The integration of rigorous data validation is also important to ensure the accuracy of results, and this includes implementing robust, well-defined guardrails to tag datasets with appropriate metadata,” he advises.
Douglas Dick, partner and head of emerging technology risk at KPMG UK, points to hallucinations occurring due to one of two factors: incomplete or inaccurate data and/or a lack of guardrails in AI models.
“If you put rubbish in, you get rubbish out,” he warns. “Hallucinations occur because the AI model does not have complete information and a set of parameters. It looks to fill those gaps with an answer because the models are based on perceived patterns.”
Sometimes these hallucinated results can be innocuous. Dick highlights an example of a fast-food chain appearing to offer beef burger ice cream. But in other cases, the results could be life-threatening, such as if a medical chatbot advised a patient to take a drug to which they were allergic.
“With any gen AI-created answer, it is important to verify and corroborate all responses with reliable or authoritative sources and maintain professional skepticism when assessing their accuracy,” Dick adds.
AI hallucinations: The rise of RAG
One way for companies and enterprises to deal with hallucinations is to embed retrieval augmented generation (RAG) into their LLMs. This taps into relevant, contextual, and up-to-date information from external sources – such as breaking news, public policy documents, or scientific resources – to deliver a factually correct response.
Dominic Wellington, enterprise architect at SnapLogic, says: “AI developers and business implementers must ensure their generative AI tools are providing responses based on accurate and reliable data.
“For instance, if I asked my generative AI tool to analyze my sales figures in the last year, does it easily have access to this data, is it connected to my analytics tool, and most importantly, am I sure that the original data is accurate? If you can’t guarantee these things, you’re likely not going to be able to trust the output.”
He adds: “RAG uses a specialized database to provide a source of ground truth that is founded on an organization’s own data, but without having to share sensitive information with outside AI models. The LLMs are used to provide the conversational interaction and language parsing capabilities that are the hallmark of generative AI applications.”
Mark Swinson, enterprise automation specialist at Red Hat, explains how the models behind generative AI are essentially based on statistics, and this means “they calculate the probabilities of a word or phrase coming next as they build a response to an initial prompt”.
“If there’s a ‘wrinkle’ in the probabilities encapsulated in the model, based on its training, then the response generated can easily diverge from facts and even from the data used to train it,” he suggests.
Swinson notes a three-pronged approach to reducing hallucinations:
- Use a model trained on a more focused data set for a specific task – sometimes these are referred to as small language models.
- “Fine-tune” LLMs with additional training data that adjusts the model to account for new information such as Red Hat and IBM’s InstructLab tool.
- Perform some validation of model outputs by automatically generating several related prompts via an LLM then comparing outputs for correlation.
However, there is one other suggested route according to Kev Breen, a former military technician who is now senior director cyber threat research at Immersive Labs.
“Generative AI hallucinations are a major risk impacting the wide use of publicly available LLMs,” he warns. “It is important to understand that generative AI doesn’t ‘look up information’. It doesn't query a database like a search site. Instead, it predicts words or tokens based on the question asked.
“This means humans can taint the conversations, leading to a higher likelihood of an AI hallucination. For example, a simple case of asking a question like ‘Tell me when this happened’ instead of ‘Tell me if this has ever happened’ will bias an AI to generate a false statement as it uses this statement to inform its answer.
“Teaching humans how to properly interact with generative AI to ensure they recognize data is not guaranteed to be true and should be fact-checked is key.”
Jonathan Weinberg is a freelance journalist and writer who specialises in technology and business, with a particular interest in the social and economic impact on the future of work and wider society. His passion is for telling stories that show how technology and digital improves our lives for the better, while keeping one eye on the emerging security and privacy dangers. A former national newspaper technology, gadgets and gaming editor for a decade, Jonathan has been bylined in national, consumer and trade publications across print and online, in the UK and the US.
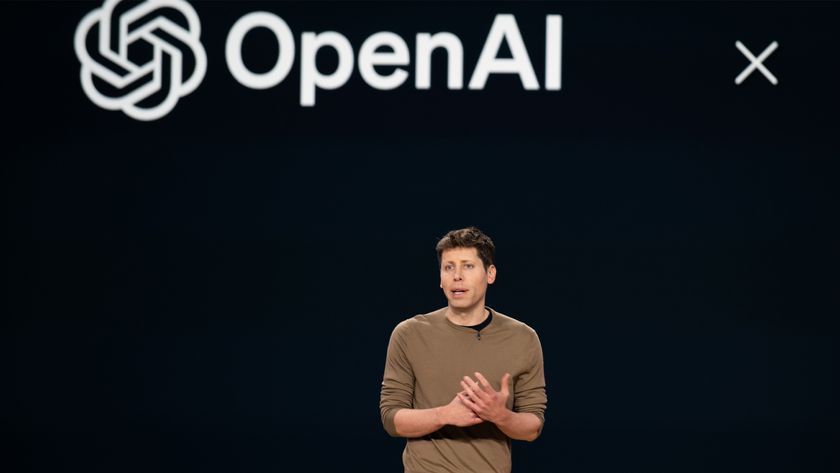
Sam Altman says ‘no thank you’ to Musk's $97bn bid for OpenAI
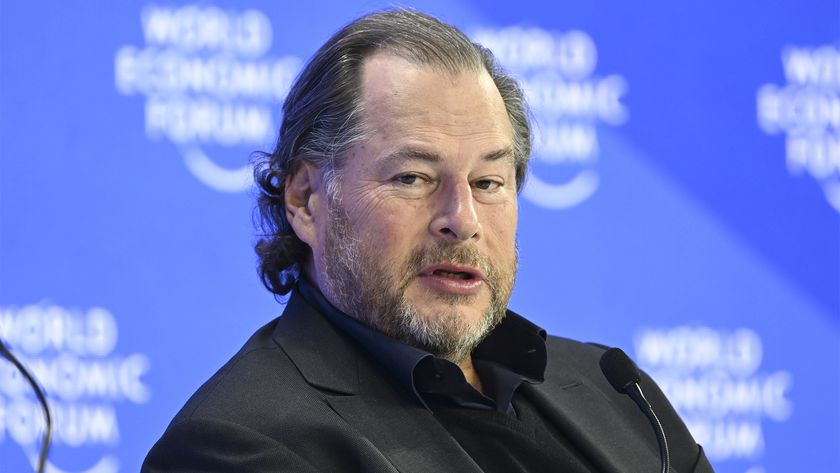
"We are really moving into a world now of managing humans and agents together": Marc Benioff thinks today’s CEOs will be the last to have a fully human workforce – and he's not the only big tech exec predicting the rise of an AI workforce