Meta executive denies hyping up Llama 4 benchmark scores – but what can users expect from the new models?
Meta has released its latest AI model, Llama 4, using a "mixture of experts" architecture
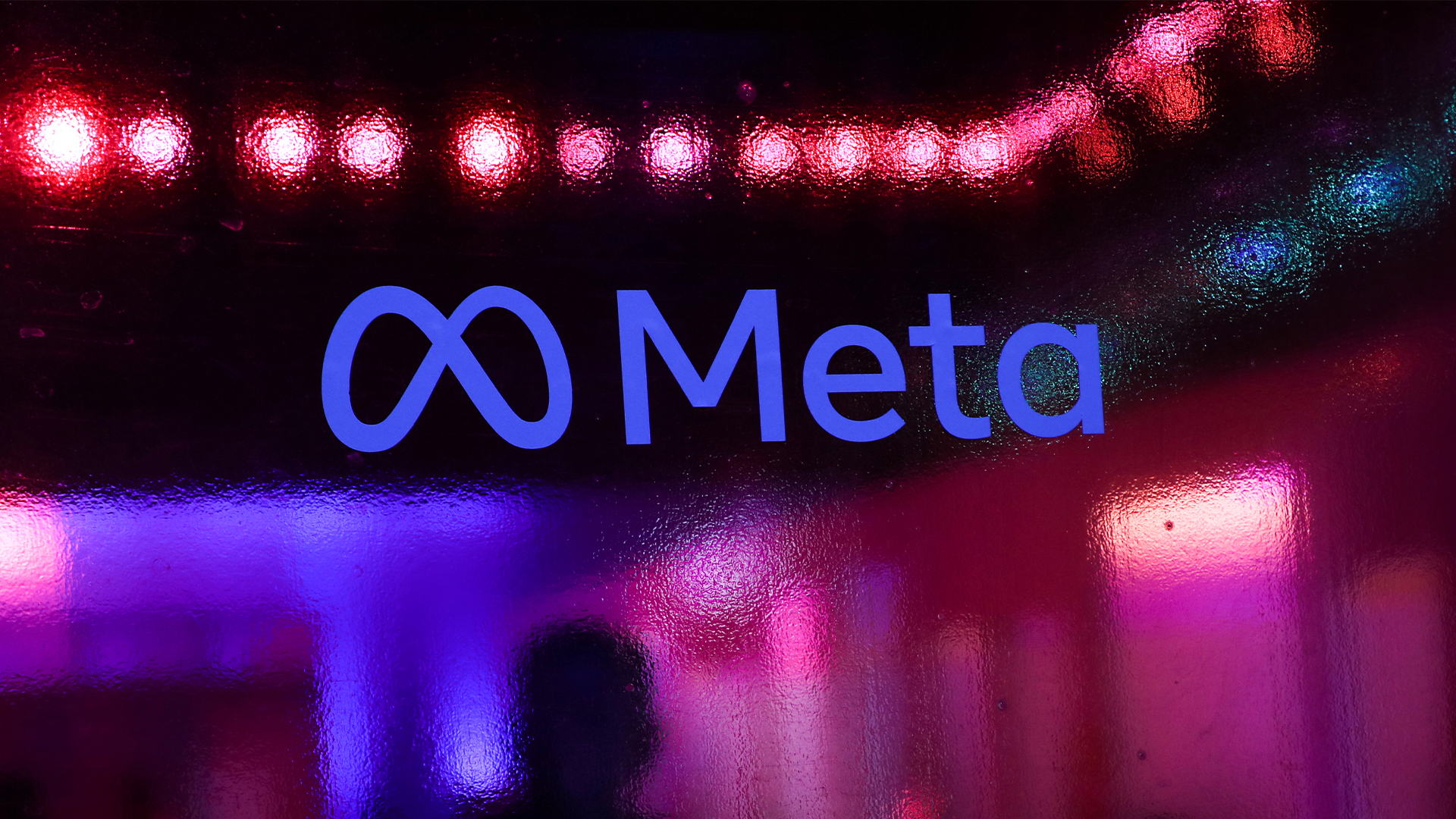
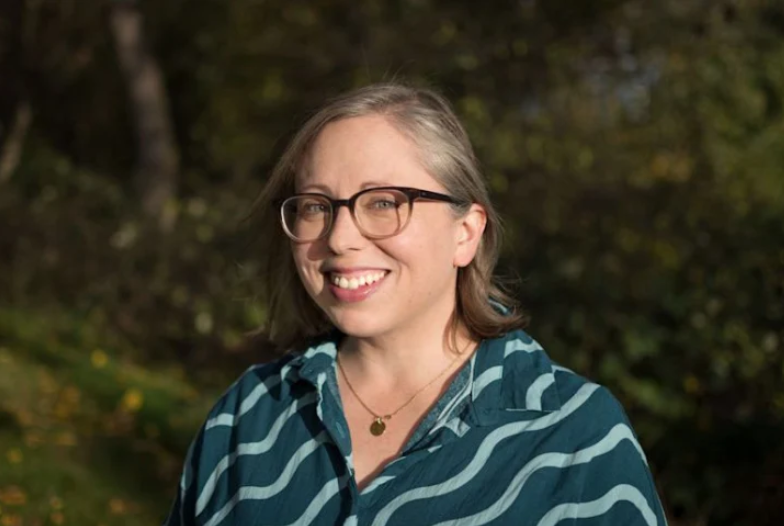
A senior figure at Meta has denied claims that the tech giant boosted performance metrics for its new Llama 4 AI model range following rumors online.
Meta’s VP of generative AI, Ahmad Al-Dahle, took to X to address the issue, claiming they’re “simply not true”. The rumors centered around claims Meta trained its new Llama 4 Maverick and Scout models on “test sets”.
These data sets are used to evaluate model performance post-training. The speculation over Meta’s alleged use of test sets suggested this would make the model appear more powerful than it is compared to rival options.
This was further fueled by posts online that the Maverick and Scout models have performed below expectations, particularly when used with different cloud service providers.
Al-Dahle admitted that some early users have experienced “mixed quality”, but said the company expects it will take time for public implementations to reach maximum output and for wrinkles to be ironed out.
“We've also heard claims that we trained on test sets -- that's simply not true and we would never do that,” Al-Dahle said. “Our best understanding is that the variable quality people are seeing is due to needing to stabilize implementations.
“Since we dropped the models as soon as they were ready, we expect it'll take several days for all the public implementations to get dialed in. We'll keep working through our bug fixes and onboarding partners.”
Get the ITPro daily newsletter
Sign up today and you will receive a free copy of our Future Focus 2025 report - the leading guidance on AI, cybersecurity and other IT challenges as per 700+ senior executives
The speculation over model performance juicing may have put a dampener on the highly anticipated launch of the Llama 4 model range, which is powered by a massive 288-billion parameter "teacher" model called Behemoth.
These new models use a "mixture of experts" format and include a teaching model, which are designed to overcome scaling challenges such as the cost of simply making bigger models.
In this instance, Behemoth has 288 billion active parameters, with two trillion total parameters, and 16 experts, and is a "teacher model" that is distilled to inform the two smaller models, Maverick and Scout.
Controversy aside, Meta appears confident that the new model range will place it in good stead to compete with industry rivals - so what can users expect?
Everything you need to know about the new Llama 4 models
Llama 4 Maverick, to give the model its full name, has 17 billion active parameters, 400 billion total parameters, and 128 experts.
That combination tops competitors GPT-4o and Gemini 2.0 Flash, Meta claimed, while offering comparable results to DeepSeek v3 with fewer active parameters for a "best in class" performance to cost ratio.
Meanwhile, Llama 4 Scout features 17 billion active parameters, 109 billion total parameters and 16 experts, which fits onto a single Nvidia H100 GPU, meaning it can be used without top end systems.
Meta said the Llama lineup will let developers build "more personalized multimodal experiences", noting that it's continuing to offer access to its weights — making it more open than rivals, though some have questioned if that counts as fully open source.
Scout and Maverick are available on Llama.com and Hugging Face, and Llama 4 will power Meta AI products including those in WhatsApp, Messenger, and Instagram. Llama 4 Behemoth isn't being released yet as it's still in training.
"As more people continue to use artificial intelligence to enhance their daily lives, it’s important that the leading models and systems are openly available so everyone can build the future of personalized experiences," Meta said in a blog post.
"We’re introducing Llama 4 Scout and Llama 4 Maverick, the first open-weight natively multimodal models with unprecedented context length support and our first built using a mixture-of-experts (MoE) architecture.
"We’re also previewing Llama 4 Behemoth, one of the smartest LLMs in the world and our most powerful yet to serve as a teacher for our new models."
Rise of the expert models
Meta is attempting to pair the best of scaling massive large language models (LLMs) with more specific expertise that can be run using smaller systems.
The two smaller models, Llama 4 Scout and Llama 4 Maverick, both use 17 billion active parameters but pairing that with so-called experts - which are specialised neural networks that are activated only when necessary - helps to improve models without scaling up computing cost.
This is achieved by only using the necessary parts to answer a question and ignoring aspects that aren't needed.
For example, if you needed to answer a math question, there's no reason to include systems for science or language. Scout has 16 experts, while Maverick features 128; Behemoth also has a system of 16 experts.
"Our new Llama 4 models are our first models that use a mixture of experts (MoE) architecture. In MoE models, a single token activates only a fraction of the total parameters," the blog post noted.
"MoE architectures are more compute efficient for training and inference and, given a fixed training FLOPs budget, delivers higher quality compared to a dense model."
Meta explained that for Maverick, a token is sent to a "shared expert" as well as to one of the 128 routed experts.
"As a result, while all parameters are stored in memory, only a subset of the total parameters are activated while serving these models," the post said.
"This improves inference efficiency by lowering model serving costs and latency—Llama 4 Maverick can be run on a single NVIDIA H100 DGX host for easy deployment, or with distributed inference for maximum efficiency."
Benchmarks
Meta noted that Maverick would cost between 19 cents and 49 cents per one million input and output tokens, making it roughly inline with Gemini 2.0 Flash and DeepSeek v3.1, and significantly cheaper than GPT-4o.
At the same time, it claimed to top those rivals on most benchmarks, including image reasoning, coding and language, though DeepSeek v3.1 did pip it on one reasoning benchmark and on LiveCodeBench.
A similar pattern was shown for Scout, with it topping rivals in selected benchmarks.
Benchmarks aside, Meta claimed that Scout offers an "industry leading" context window of 10 million tokens, but AI researcher Simon Willison noted in a blog post that it's not currently possible to actually get more than a fraction of that token window running.
MORE FROM ITPRO
Freelance journalist Nicole Kobie first started writing for ITPro in 2007, with bylines in New Scientist, Wired, PC Pro and many more.
Nicole the author of a book about the history of technology, The Long History of the Future.
-
Bigger salaries, more burnout: Is the CISO role in crisis?
In-depth CISOs are more stressed than ever before – but why is this and what can be done?
By Kate O'Flaherty Published
-
Cheap cyber crime kits can be bought on the dark web for less than $25
News Research from NordVPN shows phishing kits are now widely available on the dark web and via messaging apps like Telegram, and are often selling for less than $25.
By Emma Woollacott Published
-
Fake it till you make it: 79% of tech workers pretend to know more about AI than they do – and executives are the worst offenders
News Tech industry workers are exaggerating their AI knowledge and skills capabilities, and executives are among the worst offenders, new research shows.
By Nicole Kobie Published
-
Sourcetable, a startup behind a ‘self-driving spreadsheet’ tool, wants to replicate the vibe coding trend for data analysts
News Sourcetable, a startup developing what it’s dubbed the world’s first ‘self-driving spreadsheet’, has raised $4.3 million in funding to transform data analysis.
By Ross Kelly Published
-
DeepSeek and Anthropic have a long way to go to catch ChatGPT: OpenAI's flagship chatbot is still far and away the most popular AI tool in offices globally
News ChatGPT remains the most popular AI tool among office workers globally, research shows, despite a rising number of competitor options available to users.
By Ross Kelly Published
-
‘DIY’ agent platforms are big tech’s latest gambit to drive AI adoption
Analysis The rise of 'DIY' agentic AI development platforms could enable big tech providers to drive AI adoption rates.
By George Fitzmaurice Published
-
Fit for artificial intelligence
whitepaper Ensure data availability to applications and services with hybrid cloud storage
By ITPro Published
-
Fit for AI
whitepaper Ensure data availability to applications and services with hybrid cloud storage
By ITPro Published
-
'Customers have been begging us to launch': AWS just rolled out Amazon Q Business in Europe – and it includes new data residency features
News AWS has announced the availability of its Amazon Q Business platform in Europe in a move sure to please sovereignty-conscious customers.
By George Fitzmaurice Published
-
Google DeepMind’s Demis Hassabis says AI isn’t a ‘silver bullet’ – but within five to ten years its benefits will be undeniable
News Demis Hassabis, CEO at Google DeepMind and one of the UK’s most prominent voices on AI, says AI will bring exciting developments in the coming year.
By Rory Bathgate Published