Powering the AI revolution
Large language models and generative AI could change the way we work, but businesses need the right hardware to run them
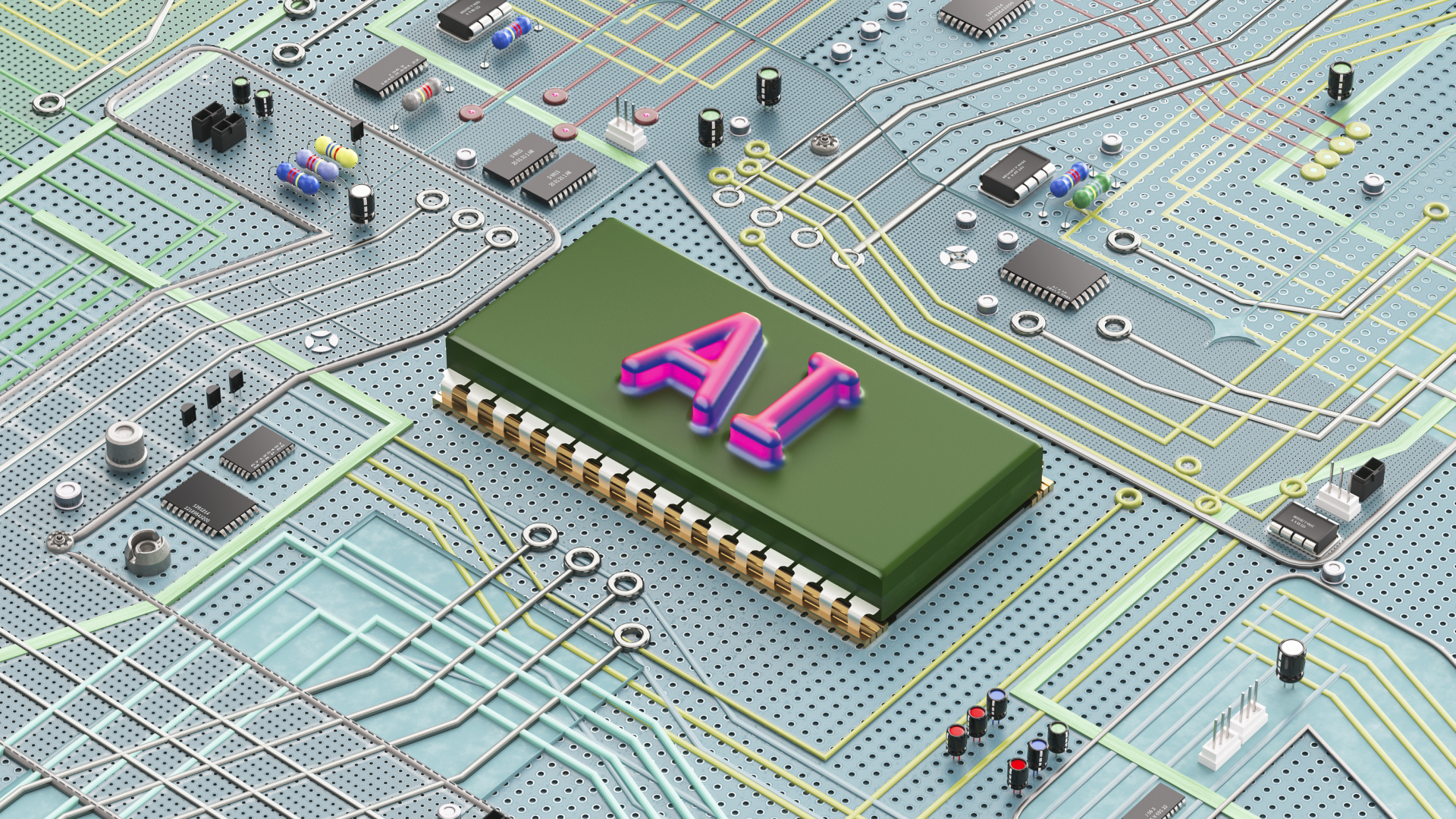
While the hype around artificial intelligence (AI) has never been higher than it is now, technologists will know that in some respects this technology is nothing new. For example, pattern recognition software has been incorporated into information security and antimalware systems for some time, looking for abnormal behavior in a network and determining if it’s likely to represent a threat.
Similarly, automation has been a part of IT and thus business as a whole for many years, eliminating the need for people to do repetitive tasks if they don’t have to. This could be anything from automated payroll to chatbots that can triage customer requests into something that can be given an automated response (which it can also handle) and those that require a human touch.
It’s easy, then, to be cynical about Johnny-come-lately generative AI chatbots and image generators. The fevered discourse around them could give the impression that we’re on the brink of a utopian society of leisure ushered in by a benign robot deity, or alternatively that a malevolent, all-powerful machine will enslave us all by next Tuesday. The reality, thankfully, is likely to be much more mundane.
However skeptical or enthusiastic someone is about the programs currently on offer, though, the technology underpinning them is something to get excited about – and something to prepare for.
Introducing the large language model
The most interesting and powerful of all the advances made in artificial intelligence in the past 10 years is the creation of large language models (LLMs).
FEATURED RESOURCE
Read how a hybrid approach to cloud architecture provides an ideal solution to fluctuations in computing resources.
These are massive artificial neural networks that are fed huge amounts of text that they process in a relatively short period of time. Having processed what may be millions or even trillions of words, the LLM is then able to generate text that reads naturalistically (as if a human had written it) and can provide answers and insights more quickly than if a person or even a team of people were to carry out the research themselves. These two parts of the process are referred to as training and inferencing.
Newer generative AI tools, such as ChatGPT, use LLMs and are built using everything their creator could scrape from the internet up until the point they were trained. For ChatGPT, that’s September 2021.
Sign up today and you will receive a free copy of our Future Focus 2025 report - the leading guidance on AI, cybersecurity and other IT challenges as per 700+ senior executives
For businesses, these publicly accessible tools may not be that useful – indeed, there are plenty of examples of individuals using ChatGPT in a way that has damaged their organization or professional reputation, such as accidentally causing a data leak or citing non-existent legal cases in front of a judge. However, more tailored tools could hold the keys to growing or even transforming their business.
While many hours of discussion and reams of column inches have been given over to LLMs – not without reason – it’s important not to overlook the other forms of advanced AI that could help businesses as well. As Matt Foley, director of EMEA field application engineering at AMD, told TechRadarPro: “The possibilities of services such as ChatGPT and DALL·E have piqued everyone’s interest. But AI has much more to offer than writing student essays and generating uncanny artwork. It can have a significant impact on industry by empowering companies through applications such as computer vision, natural language processing, and product recommendation systems.
“CIOs must now decide which approach to take when developing in-house AI-based applications to harness it effectively.”
An example of this technology in use is the growing importance of computer vision in manufacturing for construction line performance monitoring and optimization. The technology is also invaluable in the development of self-driving vehicles and can be used in medicine to detect anomalies such as tumors or bone fractures that even a highly trained and experienced doctor is likely to miss.
It’s worth keeping in mind, therefore, that LLMs aren’t the only fruit of the AI tree and, depending on your vertical, other technologies may be equally or even more important.
The importance of infrastructure
Organizations and developers whose interest has been piqued by AI should look at how they intend to both train the models and use them to acquire knowledge – known as inferencing.
Training is a high intensity activity, involving massive amounts of data and large scale parallel processing. Depending on the organization carrying out the activity and the objective of it, it may be worth investing in the infrastructure to carry it out internally, or – more frequently – it may be worth using other organizations’ hardware. Increasing numbers of vendors that have traditionally sold data center hardware are offering AI training environments as a cloud service.
FEATURED RESOURCE
Discover a data center revitalization strategy that will help you dominate competitors in your market.
It’s also possible to make use of pre-trained LLMs, which can then be fed an organization’s own data to analyze, meaning they skip the heavy lifting and head straight for the inferencing level.
For most organizations, unless they are developing an LLM themselves, it’s the inferencing point where the true value is gained. It’s also where the greatest use can be made of computer vision, natural language processing (NLP), and product recommendation systems, all of which are also important elements of the AI mix.
Even at the inferencing stage, it’s still important to choose hardware that’s truly up to the job. This means CPUs and GPUs that can handle rapid processing and are adapted for edge computing, particularly in the case of computer vision where decisions must be made in real time, such as in autonomous vehicles.
In the company’s June 2023 Data Center and AI Technology Premier, AMD CEO Lisa Su explained: “Generative AI and large language models have changed the landscape. The need for more compute is growing exponentially, whether you’re talking about training or, frankly, whether you’re talking about inference. Larger models give you better accuracy and there’s a tremendous amount of experimentation and development that’s coming across in the industry.”
AMD has a wide range of chips that can help organizations make the most of the inferencing process. These include its EPYC CPU range, which is popular in data centers worldwide for all kinds of workloads; Instinct GPUs, which are optimized for deep learning, artificial neural networks, and high performance computing (HPC), including the MI300A and MI300X accelerators, which have been specifically designed for generative AI workloads and LLMs respectively; and Xilinx Versal and Zynq adaptive systems on a chip (SoCs).
As well as being available as individual components, they can also be used as part of the company’s Unified Inferencing Frontend. This gives organizations greater flexibility in switching between the three most popular AI frameworks – Tensorflow, PyTorch, and ONNX Runtime – and allows them to adapt the mix of GPUs, CPUs, and adaptive SoCs they use as their needs evolve.
We are at the base of the generative AI and LLM mountain. The hardware advances being made now, especially at the chip level, will allow these technologies to grow in ways that are likely beyond our imagination. Along with computer vision, natural language processing, and product recommendation systems, they will open up new product types, technologies, and ways of moving about our world – providing there’s the right hardware there to underpin them.
ITPro is a global business technology website providing the latest news, analysis, and business insight for IT decision-makers. Whether it's cyber security, cloud computing, IT infrastructure, or business strategy, we aim to equip leaders with the data they need to make informed IT investments.
For regular updates delivered to your inbox and social feeds, be sure to sign up to our daily newsletter and follow on us LinkedIn and Twitter.
-
Cloudflare is cracking down on AI web scrapers
News Cloudflare CEO Matthew Prince said AI companies have been "scraping content without limits" - now the company is cracking down.
-
Swiss government data published following supply chain attack – here’s what we know about the culprits
News Radix, a non-profit organization in the health promotion sector, supplies a number of federal offices, whose data has apparently been accessed.
-
AMD Advancing AI 2025: Racks, openness, and the spectre of Nvidia
Opinion Can the chipmaker really step out of the market leader's shadow?
-
What is an AI factory and what does it mean for enterprises?
Supported This form of specialized computing infrastructure powers some of our most popular services today
-
5th Gen Amd Epyc™ Processor Architecture
whitepaper
-
Advance Your Enterprise Ai Journey
whitepaper
-
How To Get Up To 20% More Ai Performance From High-performance Gpus
whitepaper
-
Maximize Gpu Efficiency With Amd Epyctm High-frequency Processors
whitepaper
-
What is exascale computing? Exploring the next step in supercomputers
60 years after the birth of the first supercomputers, we are entering a new era
-
AMD has put in the groundwork for a major AI push while the tech industry has fawned over Nvidia
Analysis The processor giant will be keen to use its AMD Advancing AI Event in San Francisco to capitalize on recent successes