This new device could be the key to solving AI’s energy efficiency problem
A US-based research team says its computational random-access memory technique processes data entirely within the memory array
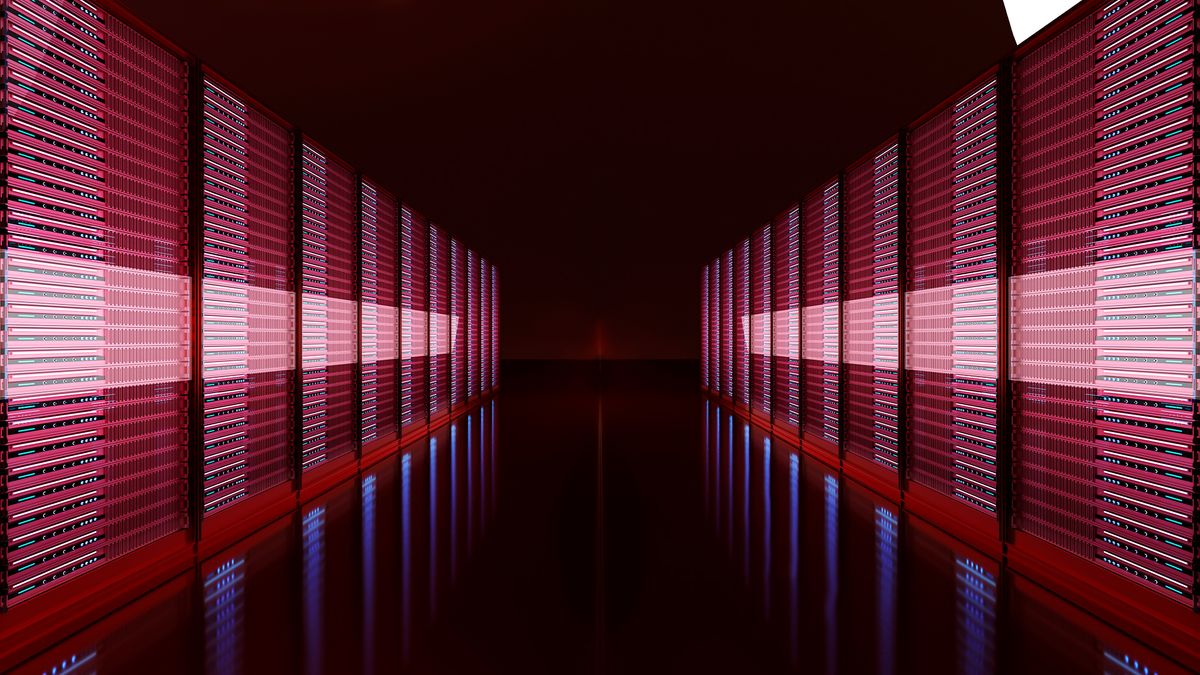
Researchers from the University of Minnesota Twin Cities have created a device they say could reduce AI energy consumption by a factor of at least 1,000.
Usually, machine learning or AI processes transfer data between logic, where information is processed within a system, and memory, where the data is stored.
This transfer consumes a large amount of power, researchers said, demanding significant resources.
But the new device keeps the data permanently in what's called computational random-access memory (CRAM). CRAM performs computations directly within memory cells, using the array structure more efficiently and eliminating the need for slow and energy-intensive data transfers.
"As an extremely energy-efficient digital based in-memory computing substrate, CRAM is very flexible in that computation can be performed in any location in the memory array. Accordingly, we can reconfigure CRAM to best match the performance needs of a diverse set of AI algorithms," said Ulya Karpuzcu, co-author on the paper and associate professor in the Department of Electrical and Computer Engineering.
"It is more energy-efficient than traditional building blocks for today’s AI systems."
Tests of the CRAM-based machine learning inference accelerator have shown an improvement in the order of 1,000, researchers noted, with other examples bringing energy savings of 2,500 and 1,700 times compared to traditional methods.
Get the ITPro. daily newsletter
Receive our latest news, industry updates, featured resources and more. Sign up today to receive our FREE report on AI cyber crime & security - newly updated for 2024.
The work extends the team's previous research into Magnetic Tunnel Junctions (MTJs), which are nanostructured devices used to improve hard drives, sensors, and other microelectronics systems, including Magnetic Random Access Memory (MRAM).
The most efficient short-term random access memory (RAM) device uses four or five transistors to code a one or a zero, but one MTJ, a spintronic device, can perform the same function at a fraction of the energy, with higher speed, and is resilient to harsh environments, says the team.
"Our initial concept to use memory cells directly for computing 20 years ago was considered crazy," said Jian-Ping Wang, senior author on the paper and a Distinguished McKnight Professor.
The researchers have a number of patents in the pipeline, and are planning to work with semiconductor industry leaders to provide large scale demonstrations.
Earlier this year, the International Energy Agency (IEA) issued a global energy use forecast suggesting that energy consumption for AI is likely to double from 460 terawatt-hours in 2022 to 1,000TWh in 2026 - roughly equivalent to the entire electricity consumption of Japan.
And just last week, the World Economic Forum (WEF) warned that generative AI systems may already be using around 33 times more energy to complete a task than task-specific software would.
Other engineers are also aiming to drive AI energy consumption down, with Nvidia claiming that its new AI ‘superchip’ can improve performance by 30 times when running generative AI services, while using 25 times less energy.
Emma Woollacott is a freelance journalist writing for publications including the BBC, Private Eye, Forbes, Raconteur and specialist technology titles.
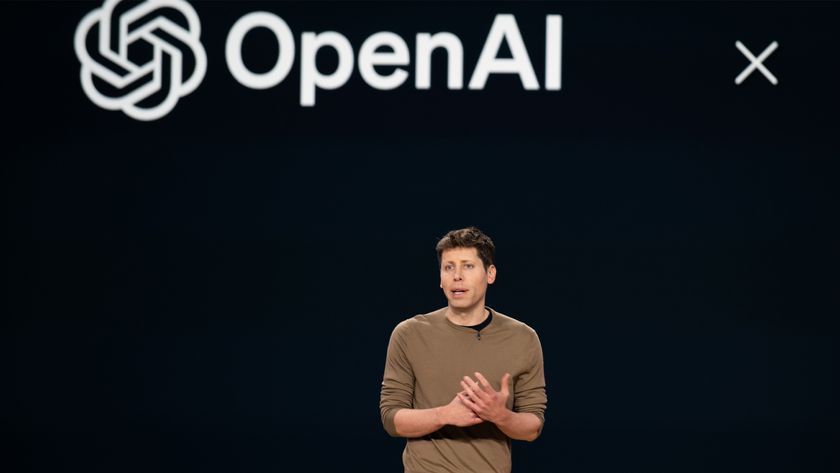
Sam Altman says ‘no thank you’ to Musk's $97bn bid for OpenAI
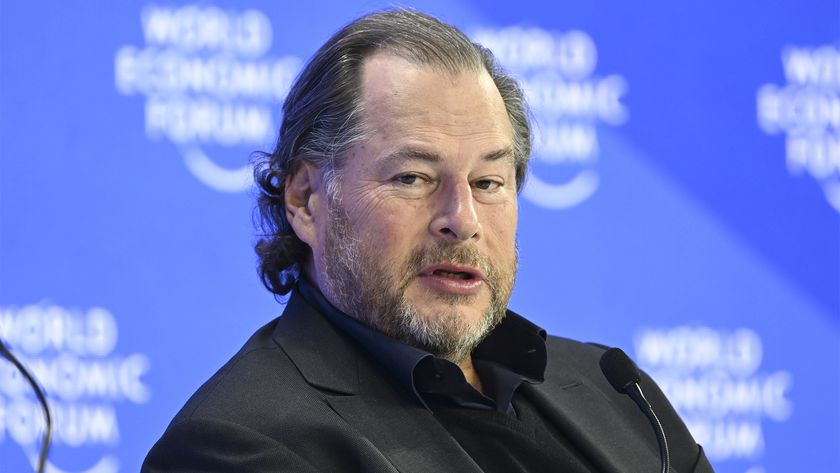
"We are really moving into a world now of managing humans and agents together": Marc Benioff thinks today’s CEOs will be the last to have a fully human workforce – and he's not the only big tech exec predicting the rise of an AI workforce