What are large quantitative models (LQMs)?
Widely used when mathematical reasoning is required, LQMs are often deployed for financial forecasting or supply chain management
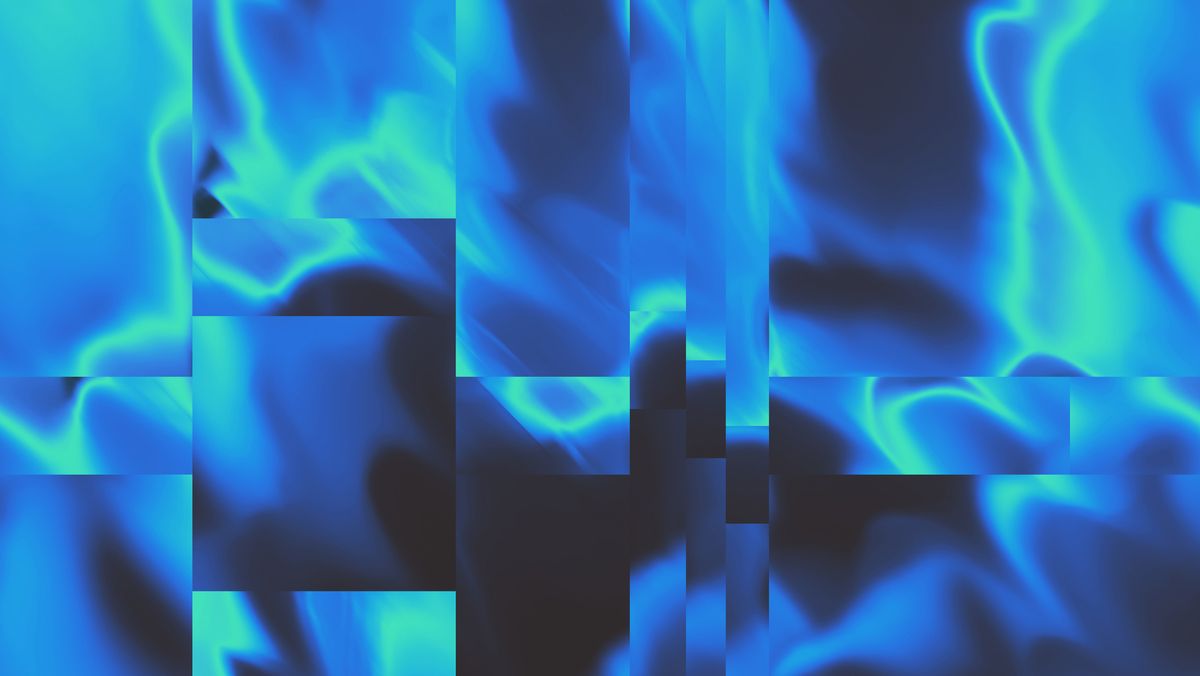
If you stay even relatively in the loop with tech news, you’ve probably heard of large language models (LLMs). They’re the backbone of generative AI, the technology currently top of mind for many business leaders at the moment.
You probably wont have heard as much about large quantitative models (LQMs), the LLM’s more mathematically-minded sibling. Where LLMs are great at generating creative outputs, LQMs are more attuned to statistical analyses and mathematical forecasting.
They are large, specialized models based on numerical structure data according to Informatica’s chief architect Siddharth Rajagopal, used for providing statistical outcomes to particular use cases and problems.
“LQMs are all about math. They work by applying mathematical, statistical, or physics-based methods to structured numerical data, generating predictions or insights about complex systems,” Ian Quackenbos, AI lead at SUSE, tells ITPro.
The potential upside for businesses is high, with the potential of LQMs ranging from more effective financial forecasting to potential efficiency gains in areas like supply chain or stock management.
How do LQMs work?
LQMs work by combining the flexibility and processing power of AI with reliability and accuracy of numerical analysis according to Dr Stefan Leichenauer, VP of engineering at SandboxAQ.
For businesses to drive value, they need to explore directions that they have not previously gone, Leichenauer tells ITPro. But AI only knows what it’s been trained on so innovation can be unreliable.
Get the ITPro. daily newsletter
Sign up today and you will receive a free copy of our Focus Report 2025 - the leading guidance on AI, cybersecurity and other IT challenges as per 700+ senior executives
“On the other hand, we have fundamental quantitative principles and equations that are always valid and correct in every circumstance, and we can use those to systematically address new areas with confidence,” Leichenauer says.
An LQM blends the ability of a generative AI system to synthesize outputs with the logical accuracy of a numerical system, meaning users teach an LQM to make statistical predictions based on mathematical logic.
"When looking in a new direction, we can use understood, advanced simulation techniques to generate a new data set to augment the data that we already have. Then we give that data to an AI to teach it how to understand that new direction. The AI can then effectively explore a larger domain, and we iterate the process in a virtuous cycle to get to where we want to go,” Leichenauer said.
How do they differ from LLMs?
The main point of differentiation between LQMs and LLMs is the data that they are trained which, in turn, defines the output they generate. Where LLMs are trained on unstructured data sets, LQMs are trained on structured data sets.
LLMs are more useful for conversational interactions or the generation of content because they learn by making connections between data types like text and imagery, Rajagopal tells ITPro, while LQMs are used for mathematical inferences.
“LQMs focus on numerical and quantitative analysis, processing structured data like statistics or experimental results to make predictions or simulate systems. In contrast, LLMs specialize in understanding and generating human language, working with unstructured text data such as articles or conversations,” Quackenbos said.
“Their goals and input types are fundamentally different—LQMs excel in numbers, while LLMs thrive in words,” Quackenbos added.
Business use cases for LQMs
Before using an LQM for a mathematical task within a business, users should first consider whether a different, more traditional process could be applied, Rajagopal suggests. For example, problems that have a smaller number of parameters could be solved via a simpler machine learning (ML) model.
LQMs are useful when your business is trying to solve high-value critical problems that are not language based, Leichenauer said. Such business issues are often ones that have some bearing on the real world.
This could be the modelling of a physical process, the engineering of a new device, the optimization of logistics, or finance processes, he added. Such problems require large and complex amounts of data for which there is a clear definition of right and wrong.
An LQM can tackle these kinds of problems by combining content production with verifiable accuracy. Examples include drug discovery and precision medicine, battery optimization and materials design, and fraud detection and risk modeling in the financial domain. LQM-powered insights in these areas can accelerate R&D and improve results,” Leichenauer said.
How can LLMs and LQMs be used together?
Both types of model have their strengths and they can be combined to to work collaboratively together. This can be particularly helpful when the statistical or numerical analysis of an LQMs needs to be put into context according to Dr Juan Bernabé-Moreno, Director of IBM Research.
In areas like financial markets and trading an LQM can be used to perform complex technical analysis while and LLM can act as an interface and help break down the results of this analysis, Bernabé-Moreno told ITPro.
“LLMs excel at language-based tasks—content creation, summarization, customer interaction, and automating certain business processes. They are also useful as user interfaces for other systems, including LQMs, increasing accessibility,” Leichenauer said, echoing this sentiment.
Both LLMs and LQMs have their place in the current business landscape, though businesses should make sure they’re using them for the correct processes. To get the most out of these tools, businesses should leverage their relative strengths and, where possible, use the two types of AI to complement one another.
George Fitzmaurice is a staff writer at ITPro, ChannelPro, and CloudPro, with a particular interest in AI regulation, data legislation, and market development. After graduating from the University of Oxford with a degree in English Language and Literature, he undertook an internship at the New Statesman before starting at ITPro. Outside of the office, George is both an aspiring musician and an avid reader.