What does a data center look like in the AI era?
The AI boom has necessitated major changes in the architecture of data centers, take a look at how AI data centers differ from traditional cloud infrastructure, and the key features required to handle complex, energy-intensive AI workloads
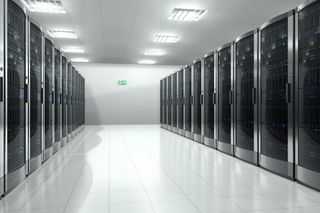
Artificial Intelligence (AI)I has been a hotbed for innovation, promising to transform industries and drive new efficiencies. As such, the infrastructure that supports these advancements has never been more critical. But AI places vastly different demands on this infrastructure, precipitating a transformation in how our data centers are designed.
Traditional data centers are CPU-centric, providing flexible processing power suitable for a number of different computational tasks. These facilities underpin the majority of enterprise applications and data storage workloads, such as web services, database management, and file storage.
The advent of cloud computing forced data centers to become more flexible and efficient such that they could provide compute power for cloud services on demand and with low latency.
The popularity of AI technologies has ushered in a new era for the data center, however, and the workloads these facilities need to compute have become far more complex and power-intensive.
Generative AI relies on training foundation models and performing inference tasks, which requires the ability to perform calculations on vast datasets quickly. This is something GPUs are far better at than CPUs and so the data center has had to evolve once more.
The architecture at the heart of the data center has changed to adapt to the requirements of AI workloads. The CPU-powered racks that previously dominated the data center are replaced with high-performance clusters composed of specialized GPUs such as the Nvidia H100, which provide the power density required to meet the computational demands of AI.
These GPUs are also complemented by hardware accelerators such as neural processing units (NPUs) and tensor processing units (TPUs), which are other types of computational chips designed to tackle complex problems involved in AI training and inference.
Get the ITPro. daily newsletter
Receive our latest news, industry updates, featured resources and more. Sign up today to receive our FREE report on AI cyber crime & security - newly updated for 2024.
These chips are particularly suited to processing large amounts of data simultaneously, with the TPU designed to quickly process machine learning algorithms, and NPUs catering to deep learning neural network operations.
AI systems need access to large amounts of unstructured data to provide high-quality outputs which require new data storage technology as well. AI data centers need higher capacity storage solutions, which also provide low-latency access to data to enable quicker training and inference times.
For example, object storage is a popular approach for storing large amounts of unstructured data, as it is easily scalable as storage requirements grow and it also allows each data point to be stored as an object with a unique identifier.
Keeping up with the energy intensity of AI
This shift in architecture reflects a step change in power density contained within AI data centers, which also necessitates new cooling techniques such as liquid and immersive cooling. Liquid cooling has become something of a must-have for AI data center operators, as traditional cooling methods struggle to manage the level of heat being generated by GPU clusters.
A survey conducted by The Register found more than a third (38.3%) of enterprises expect to employ some form of liquid cooling infrastructure in their data centers by 2026.
AI data centers typically require at least 60 kW per rack, whereas traditional data centers average somewhere in the region of 5 – 10 kW per rack, which illustrates the scale of the new power demands AI workloads are putting on data centers.
Ensuring this power is available is a challenge for operators, who have often opted to commission entirely new greenfield sites near a reliable and cost-effective source of energy instead of retrofitting traditional data centers in less advantageous locations.
Operators also need to be wary of what kind of energy generation they use to supply the power-hungry GPU clusters in their data centers. A number of major players in the cloud infrastructure industry are buying up entire renewable energy generation plants to power their facilities, to ensure they have a cheap supply of clean energy that won’t negatively impact their ESG goals.
Others have begun signing multi-year power purchase agreements (PPAs) with green energy providers to guarantee the supply of carbon-free energy for decades. This is particularly important in giving them the headroom required to keep up with the skyrocketing demand for AI infrastructure.
Scalability is a key concern when designing modern AI data centers, so the facility can add the additional processing power and storage capacity required to meet growing demand and expanding datasets. Additionally, being able to dynamically adjust and reallocate resources according to fluctuating AI workloads helps data centers manage costs more effectively.
The three types of AI data center
Today, AI data centers can be broken down into three primary categories. The biggest facilities are constructed by major organizations to train foundation models and large deep learning neural networks that can be applied to a variety of different AI use cases. These projects are usually model vendors, internet service providers (ISPs), and telecommunications companies.
Slightly less gargantuan AI data centers are used by large enterprises to train their own industry-specific models based on these foundation models, as well as to do the inferencing required once the model is up and running.
A class of smaller data centers are deployed near production centers and populations they serve to deliver inference and fine-tuning with reduced latency.
Edge computing is becoming increasingly critical in supporting AI-driven applications including robotics, autonomous vehicles, and IoT, and the proximity of edge data centers to the end-users can help minimize latency and allow faster data processing, essential for real-time analytics.
Compared to training models, inference is relatively less computationally intensive but these edge data centers still require efficient and reliable infrastructure that can deliver insights in real-time.
Overall, the scalability, computational power, and efficiency of data centers are the competitive edge for enterprise AI. Thus maximizing these areas in your data center will be key to capitalizing on surging demand for AI.
Solomon Klappholz is a Staff Writer at ITPro. He has experience writing about the technologies that facilitate industrial manufacturing which led to him developing a particular interest in IT regulation, industrial infrastructure applications, and machine learning.