Why edge AI has significant business potential
Lower latency and better security controls have to be balanced against more limited outputs
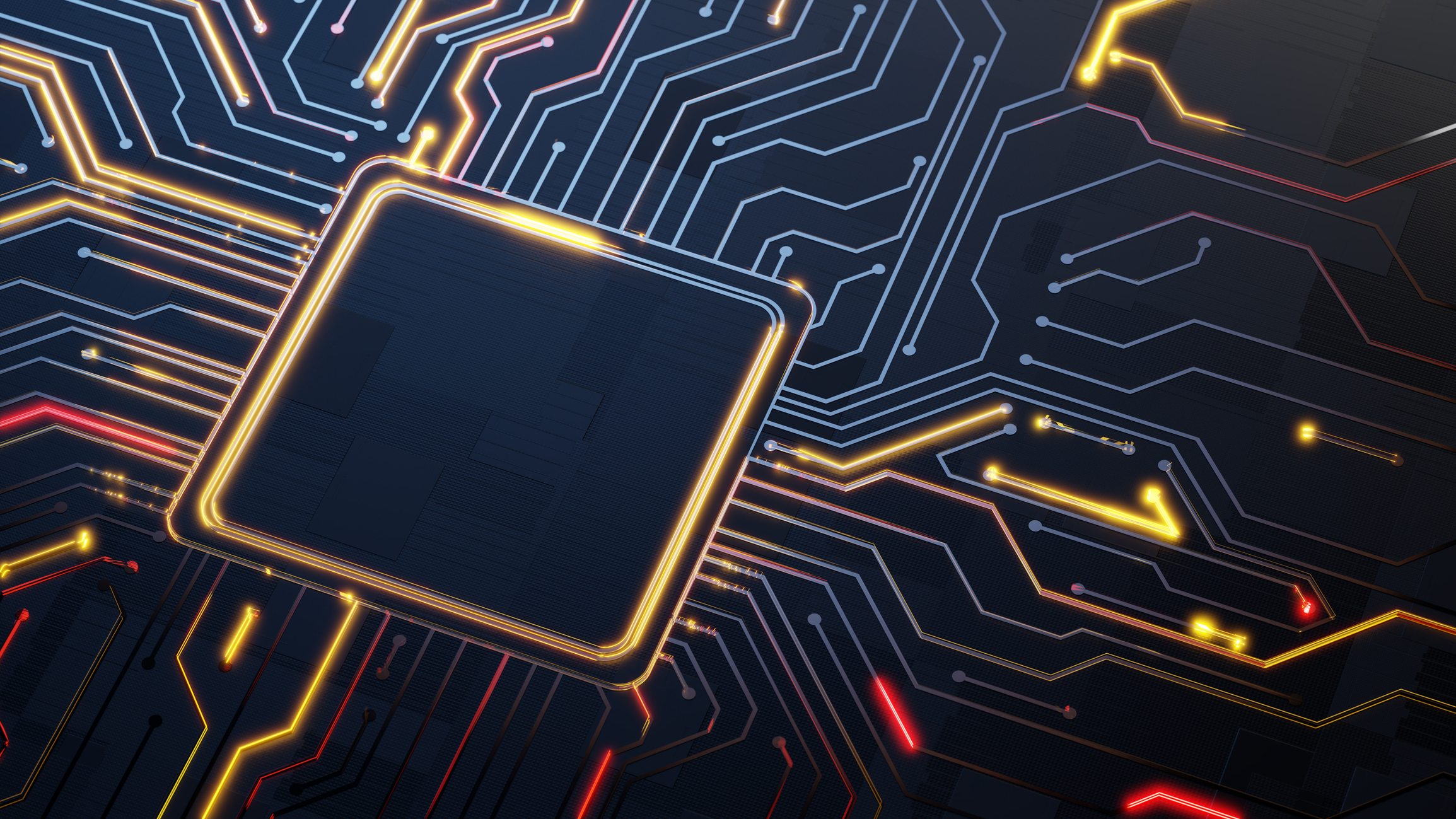
The rapid evolution of tech, and especially AI, has brought us to the era of edge computing, an approach in which data is processed closer to its source rather than relying entirely on distant cloud servers.
At the forefront of this trend is 'edge AI', where AI models and algorithms are deployed on local devices to deliver immediate insights and actions. The approach is being fueled by advancements in efficient large language models (LLMs) such as DeepSeek, as well as small language models, so-called tiny AI, and the integration of neural processing units (NPUs) in modern hardware.
For businesses, edge AI promises not just technical sophistication but tangible benefits like reduced costs, faster operations, and stronger data security.
Edge AI has the potential to redefine how businesses harness AI by moving processing power to the edge – on devices such as Internet of Things (IoT) sensors, smartphones, and industrial machines – eliminating the latency that comes with sending data back and forth to cloud servers. For example, in a smart manufacturing environment, edge AI can analyze sensor data on-site, identifying potential equipment failures before they happen.
Another critical advantage of edge AI is enhanced security. Traditional cloud-based AI requires data to travel to remote servers, introducing potential vulnerabilities along the way. In contrast, edge AI processes data locally, reducing exposure to breaches or unauthorized access, something to please IT and cybersecurity departments.
As Leo Gebbie, principal analyst & director, Americas at CCS Insight points out, “On-device AI is far more secure, given data doesn’t need to move to and from data centers, and this is seen as crucial in encouraging users to allow AI to handle more personal and private data moving forward.”
Beyond these technical and operational benefits, edge AI also can offer significant cost savings for businesses, as running AI models on local hardware can drastically reduce expenses associated with cloud storage and energy usage. Edge AI isn’t just a technological advancement; it’s a strategic shift that empowers businesses to operate smarter, faster, and more securely in an increasingly connected world.
Get the ITPro daily newsletter
Sign up today and you will receive a free copy of our Future Focus 2025 report - the leading guidance on AI, cybersecurity and other IT challenges as per 700+ senior executives
For all these reasons, edge AI is becoming more of a topic for IT decision-makers. Grand View Research estimated the total market value of edge AI as $20.87 billion in 2024, projecting a compound annual growth rate of 21.7% between 2025-30 for the market.
Enabling edge AI with small language models
Small language models and tiny AI are transforming the way AI operates at the edge.
Unlike their larger, cloud-based counterparts, these lightweight models are designed to run efficiently on devices with significantly less computing power, making them ideal for real-time applications. These models can often run on business-grade devices and even the upper end of consumer hardware such as mid-range graphics processing units (GPUs).
A good example is small language models that power on-device virtual assistants, enabling voice recognition and translation services without sending data to the cloud. By keeping all processing local, the models enable faster response times, greater reliability in areas with poor connectivity, and enhanced privacy as sensitive data remains on the device.
Deploying these efficient models, companies can reduce operational costs associated with cloud infrastructure while improving service quality. In retail, for example, edge AI can analyse in-store customer behavior to personalise recommendations instantly, while in logistics, it can optimize delivery routes in real time.
AI PCs and NPUs
The rise of AI PCs and the inclusion of NPUs in modern hardware have been pivotal developments in bringing AI down from the cloud and to the edge.
NPUs are specialised chips designed to handle complex AI workloads, enabling devices to process tasks like image recognition, natural language processing, and predictive analytics in tandem with other processes handled by the device’s CPU and GPU. This hardware advancement is particularly significant in industries requiring real-time responsiveness, such as manufacturing or health care, where latency can mean the difference between success and failure.
But it’s important to note that NPUs aren’t entirely necessary for edge AI. Gebbie tells ITPro that these chips are still optional if the goal is simply to inference data locally.
“The presence of an element such as an NPU isn’t a prerequisite to a device qualifying as an AI PC or AI smartphone,” he says. “Lots of devices are capable of running AI on-device – perhaps by using their CPU and GPU – and we define AI devices by their ability to run software, rather than by hardware.”
While NPUs provide a performance boost, many businesses are leveraging existing CPUs and GPUs to run AI models effectively, making edge AI more accessible without requiring a complete overhaul of infrastructure. For example, laptops and desktops with AI capabilities can handle tasks like fraud detection in banking or automated customer support in real time, streamlining operations.
Business applications and challenges for edge AI
One key advantage of edge AI is the ability to lower operational expenses by running AI models on local hardware rather than relying on cloud systems which charge per input and output.
Latency reduction is another critical benefit of edge AI, especially in environments where immediate responsiveness is essential. On a smart factory floor, edge AI can enable machines to detect and respond to anomalies within milliseconds, ensuring uninterrupted operations.
But despite its advantages, edge AI does present several challenges for enterprises.
Implementing edge AI solutions often requires significant upfront investment in hardware and software, which can be a barrier for smaller businesses. While mid-range devices may be technically capable of running an AI model, leaders will have to weigh up whether these cheaper options deliver the speed and performance desired – and fork out for more expensive options if they’re seeking to run the most sophisticated edge models.
RELATED WHITEPAPER
Edge AI systems may also lack the scalability and raw computational power of cloud-based models, limiting their ability to process complex datasets or accommodate rapid growth. It’s for this reason that IT leaders may choose a blended approach, designating tasks that need low latency to edge AI models while relegating those that can take more time or require far more data to be examined to their cloud workloads.
A necessary trade-off with edge AI is accepting that models small enough to run locally are, at present, less powerful than the leading LLMs. For example, DeepSeek and the smaller of Meta’s Llama models can be run locally but neither score as high as OpenAI’s reasoning models or the latest of Google’s Gemini releases.
The future of edge AI seems poised to bring even greater leaps forward, with the development of specialised AI models tailored for edge computing environments. These models, designed for efficiency and precision, will enable more industries to adopt edge AI, particularly as 5G and IoT continue to expand.
Emerging applications like autonomous vehicles, augmented reality, and real-time supply chain optimization are already benefiting from edge AI’s ability to deliver low-latency performance. Innovations in AI hardware, such as more advanced NPUs and hybrid processing capabilities, will further enhance the speed and scalability of edge AI solutions.
As edge AI evolves, its impact on industries like manufacturing, health care, and retail is expected to grow exponentially. For businesses, the case for adopting edge AI is becoming increasingly compelling. While initial investments in hardware and system integration may be high, the long-term benefits – reduced reliance on the cloud, operational agility, and the ability to innovate rapidly – far outweigh the challenges.
As edge AI continues to mature, businesses that embrace this technology will be well-positioned to lead in their respective industries, leveraging the power of localised intelligence to drive growth and sustainability.