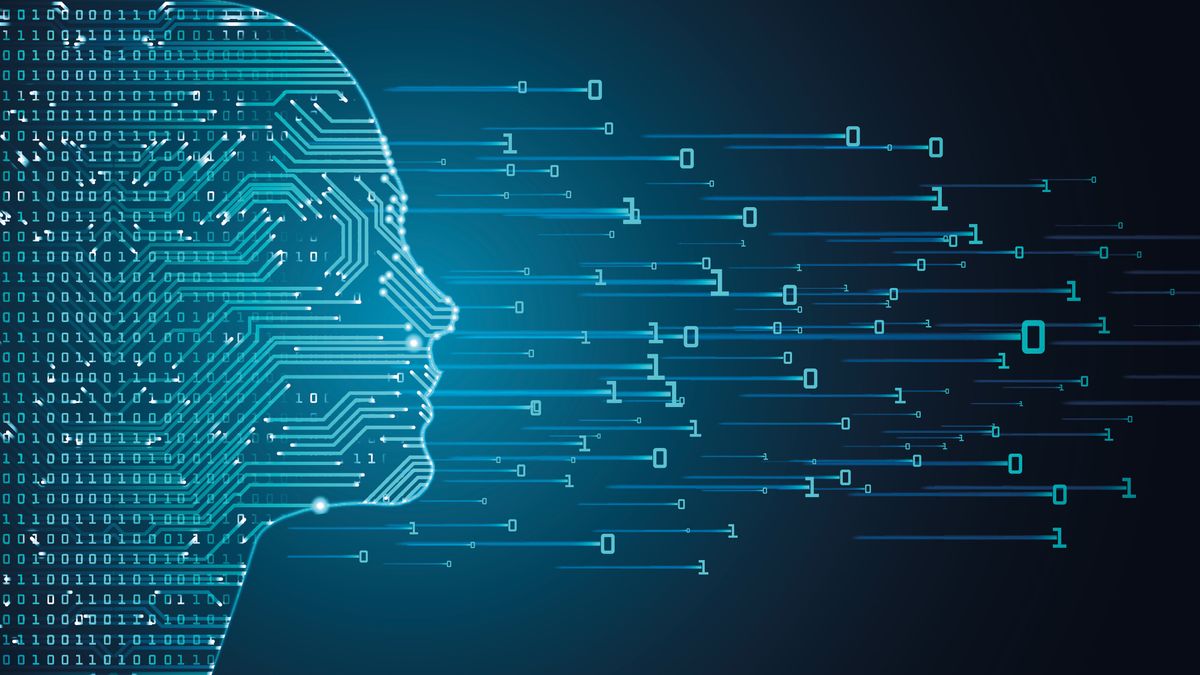
Amazing things happen when different organisations work together. It’s something that we see in business and technology, where collaboration has often helped drive new ideas or product categories forwards, and something we’ve seen over the last year or so in medicine and science, as scientists, institutions and pharmaceutical companies have worked together to fight the COVID-19 pandemic. Now, though, collaboration could also prove crucial to harnessing the power of machine learning and AI, in turn fuelling further developments in medicine, business, technology and science, but only if organisations can find a secure way to share data. To be more specific, they need a way for their machine learning models to train using data from a wider range of datasets, while reducing the risk of compromising the privacy or security of the data.
Machine learning is already revolutionising fields as diverse as finance, security, public services, manufacturing and transportation. It’s helping doctors to spot and diagnose conditions, fraud investigators to uncover money laundering and city transport planners to optimise their transport systems. But before machine learning models can analyse streams of data and a problem or recommend an action, they need to be trained using existing datasets. Generally speaking, the more data they have to work with, the more accurate and useful their models will be.
In some cases, one organisation will have access to all the necessary data, but in many others, it might be advantageous – even critical – to have access to further datasets. In healthcare, smaller institutions with limited datasets could benefit from access to training data from larger institutions. Or, in research on rare diseases, no one institution might have access to a large enough pool of data.
Sharing datasets makes sense, as every organisation involved could benefit from the resulting model. As Walter Riviera, AI Technical Solution Specialist for Intel puts it, ‘Think of it in a COVID scenario. All the hospitals in the world have COVID datasets, and it would be amazing if, in such tough times, all the hospitals in the world would agree to share their knowledge and learning and build an artificial intelligence algorithm to help beat COVID in new ways.’
Sadly, it’s not so easy. Capturing, storing and preparing data costs money, and the data itself has value to the organisation that holds it. This is an issue everywhere, but especially in operations where, say, a range of financial institutions have a shared interest in training models to combat fraud, but can’t be expected to share valuable business data with rival organisations.
As Riviera says, ‘we now appreciate the important of sensitive data and the need for regulations like GDPR, while there is private information that belongs to a specific company or brand that needs protection. Yet it would be great if we could take advantage of that information, because it’s such a valuable resource.’
The solution is federated learning. Riviera talks about it in terms of ‘an agreement between institutions’ allowing you to train a single, unified model, common to all the institutions, but trained locally by each institution. However, with each iteration of training, the model is shared with an entity called the aggregator, which takes new contributions from each model, merges them together into a new single model, then pushes that model back to all contributors. The data itself isn’t shared.
However, to make federated learning work, it needs to be secure. Those involved need to feel that any data they’re using to train the model is protected, as is the model itself. Why is this important? Because, as Walter Riviera explains, ‘One of the attacks we expect with federated learning is people trying to infer the nature of the original data by looking at the model training. If you can intercept the model at a given timestamp and you are also able to intercept that model after one iteration, then there’s a possibility that you could reverse engineer and infer what the original data was according to the update.’
This means that institutions need to be able to trust that any data being processed is protected while in use for training from others inside the programme or external actors. This requires a layer of confidentiality and security baked in at the fundamental hardware level.
This is where Intel® Software Guard Extensions (Intel® SGX) technology comes in. Built into and enhanced for the latest 3rd generation Intel® Xeon® Scalable processors, it creates a protected environment, or enclave, in system memory, where the most sensitive processes in an application can work on the most sensitive data. What goes on in the enclave is encrypted and decrypted once inside the processor core, and isolated from other processes or applications, the OS and the hypervisor. What’s more data produced and released from the enclave is sealed and run through an attestation process, to help to ensure that it hasn’t been modified.
‘In the case of federated learning, what Intel SGX allows us to do is to protect the model training’ says Walter Riviera. ‘It can help to ensure that training happens within a locked environment.’ While attacks on data during processing are comparatively rare, there are still over 11,000 potential vulnerabilities in the Common Vulnerabilities and Exposures (CVE) database, that might allow an attacker to compromise data while in use. By isolating processes and data from the OS and virtual machine software layers, Intel® SGX provides an extra level of protection from these attacks. And because the training model and data are secured by Intel® SGX, there’s an even smaller possibility of anyone being able to infer the original data. As Riviera puts it, federated learning ‘is not touching the data, it is working on how the model evolves, so it’s really critical to be able to protect the environment where the model gets trained. With Intel® SGX we can offer that capability.’
There are already great examples of federated learning and Intel® SGX at work. Intel Labs and the Perelman School of Medicine at the University of Pennsylvania are co-developing technology that empowers a federation of 29 international healthcare and research institutions to train ML models to identify brain tumours. With Intel® SGX, each institution can use patient data, including medical imaging, to train the unified model, safe in the knowledge that their data is encrypted while in use. The group believes that federated learning will accelerate the development of new ML models that will identify brain tumours earlier and more accurately.
Meanwhile, Intel® is working with Consilient, a new player in the fight against money laundering and the financing of terrorism. Consilient uses a new federated learning platform, powered by Intel® SGX, that enables financial institutions to collaborate on training ML models that might help battle these criminal activities, which involve up to 5% of global GDP every year.
What’s more, this is only the start. Across many industries and sectors, federated learning could enable more organisations to work together and build machine learning tools and models that could transform their operations. The same processes could also help individual companies as they train models using data from distributed devices or IoT devices on the edge. In recent research, AI 2.0: Upgrade Your Enterprise With Five Next-Generation AI Advances, the analyst Forrester singled federated learning out as one of five key advances that could upgrade AI to a ‘version 2.0’.
What’s more, this is only the start. Across many industries and sectors, federated learning could enable more organisations to work together and build machine learning tools and models that could transform their operations. The same processes could also help individual companies as they train models using data from distributed devices or IoT devices on the edge. In recent research, AI 2.0: Upgrade Your Enterprise With Five Next-Generation AI Advances, the analyst Forrester singled federated learning out as one of five key advances that could upgrade AI to a ‘version 2.0’.
And by providing not just the technology to accelerate AI, but the technology that helps to enable federated learning in a secure, protected environment, Intel is playing a key role in building the future of machine learning and AI.
Discover more about Intel® Software Guard Extensions
Disclaimer
Intel technologies may require enabled hardware, software or service activation.
Disclaimer
No product or component can be absolutely secure.
Disclaimer
Your costs and results may vary.
Disclaimer
© Intel Corporation. Intel, the Intel logo, and other Intel marks are trademarks of Intel Corporation or its subsidiaries. Other names and brands may be claimed as the property of others.
Get the ITPro. daily newsletter
Receive our latest news, industry updates, featured resources and more. Sign up today to receive our FREE report on AI cyber crime & security - newly updated for 2024.
ITPro is a global business technology website providing the latest news, analysis, and business insight for IT decision-makers. Whether it's cyber security, cloud computing, IT infrastructure, or business strategy, we aim to equip leaders with the data they need to make informed IT investments.
For regular updates delivered to your inbox and social feeds, be sure to sign up to our daily newsletter and follow on us LinkedIn and Twitter.