We’re a step closer toward a working quantum system and neuromorphic computing – either would revolutionize tech forever
Quantum physics and the biology of the human brain offer glimpses into the future of computing – but there is a long way to go before developments move from the lab to the enterprise.
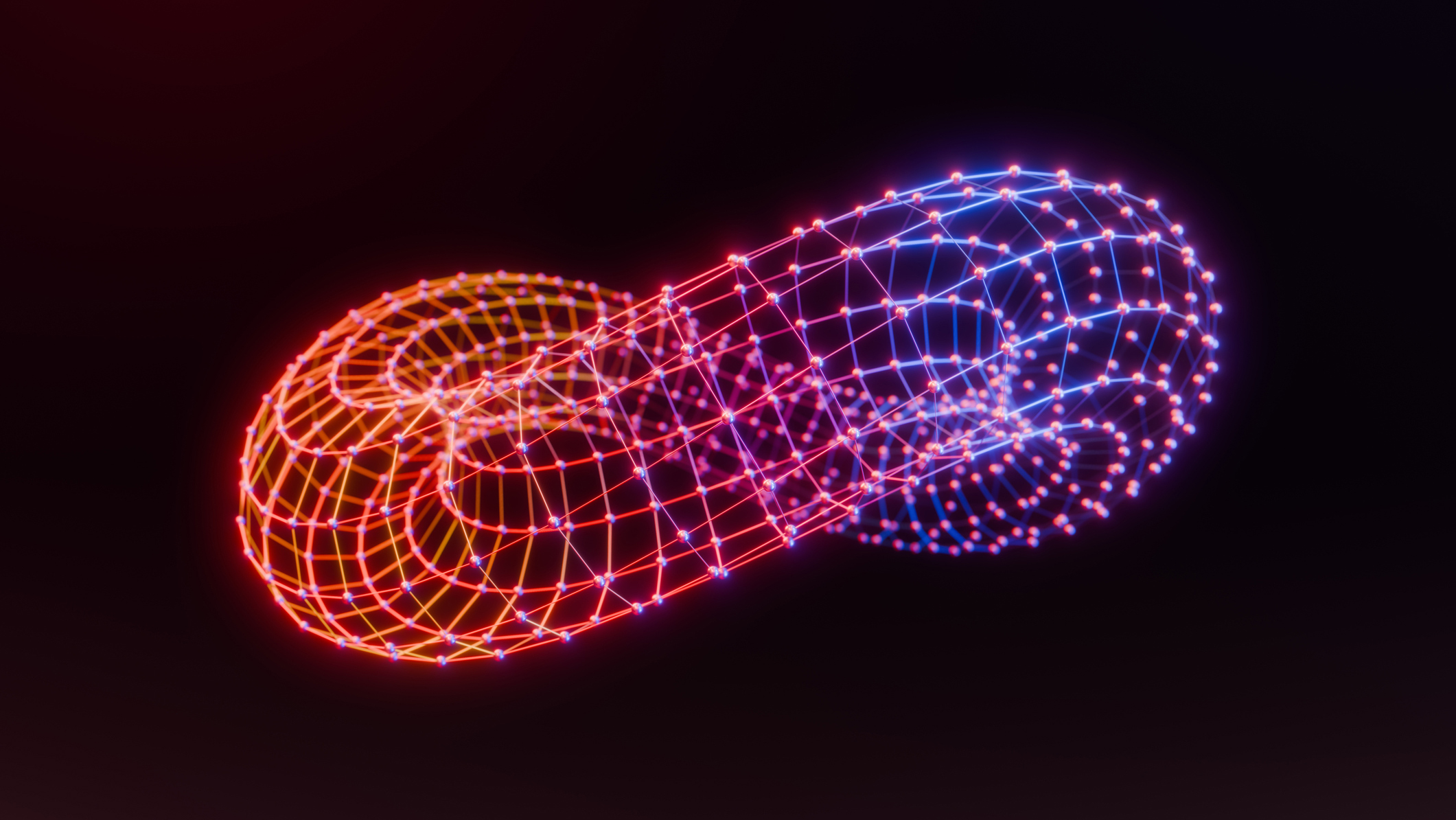
When Google announced its Willow quantum chip, there was a lot of talk about the notion that we might live in a multiverse, and of solving computational problems that would otherwise take longer than time itself. But this all seems a long way from day-to-day IT operations.
In Google’s blog post announcing Willow, founder and lead of Google Quantum AI Hartmut Neven talks about solving a “standard benchmark computation in under five minutes that would take one of today’s fastest supercomputers 10 septillion (that is, 1025) years — a number that vastly exceeds the age of the universe”.
This, Neven said, “lends credence to the notion that quantum computation occurs in many parallel universes, in line with the idea that we live in a multiverse,” and references the theoretical physicist and quantum theory researcher, David Deutsch.
What, though, does all this mean for CIOs?
These achievements, although considerable, are rather more mundane than the talk of multiverses suggests. Google says Willow goes a long way to addressing one of the practical challenges of quantum computing, error correction.
Quantum’s error correction problem
In the simplest terms, quantum computers become less reliable as they scale.
In “classical” computer systems, including supercomputers, users would expect a more powerful machine to produce more accurate results. In quantum computing, adding more qubits – a quantum system’s unit of computation – makes it more error prone, due to the physics involved. Eventually, the system becomes “classical” rather than quantum, and loses its advantages.
Get the ITPro daily newsletter
Sign up today and you will receive a free copy of our Future Focus 2025 report - the leading guidance on AI, cybersecurity and other IT challenges as per 700+ senior executives
With Willow, Google was able to add more qubits but make the system more, not less, accurate. With advances in error correction, Google achieved an exponential reduction in errors. Willow is “below threshold”: in other words Google was able to add more qubits but drive down errors. There is a full explanation of how this works, in a paper by the Google team in the journal Nature.
Neven described this as a first and vital step towards producing a usable quantum computer. “It’s a strong sign that useful, very large quantum computers can indeed be built,” he wrote. “Willow brings us closer to running practical, commercially-relevant algorithms that can’t be replicated on conventional computers.”
The next step will be a scalable architecture built around a single-qubit device called a tetron, building up to larger arrays of tetrons delivering multiple error-corrected qubits.
This is also what Microsoft is aiming for with its newly-announced Majorana 1 chip, which the firm says could enable practical quantum computing once scaled to support one million qubits. The firm is also aiming to build a prototype of a fault-tolerant quantum computer as part of a program for the US Defense Advanced Research Projects Agency (DARPA).
Both firms are aiming to throw quantum computations at the most difficult problems in science, those that even exascale systems such as the world's fastest supercomputer El Capitan can't crack.
There is, though, still much to be done.
Although Willow’s results are impressive, the computational challenge it solved is one that is optimised for quantum systems. The Random Circuit Sampling benchmark is regularly used in quantum computing research, but tells us less about a system’s real world potential.
Then there are the practicalities. Willow’s error correction is good, but not yet good enough to allow it to compete with classical computers. And Willow uses supercomputing qubits that need to be kept at temperatures Google describes as “colder than outer space”. Work still needs to be done to build systems that can fit into a conventional datacentre.
“There’s a bit of a quantum rush right now, probably because of anxiety about not seeing LLMs coming,” Jon Collins, VP of engagement and field CTO at analysts GigaOm told IT Pro.
“Nonetheless organisations can start thinking about what might be the impact on their business — it’s not to soon to work out what the heck is quantum, and where it might have useful applications.” CIOs can, for example, work with providers such as AWS that have quantum sandboxes, to test out the technology.
Collins adds that firms need to pay particular attention to quantum’s impact on encryption and data protection more generally. “While quantum computing may not be a practical reality by 2025, preparing for its impact on cybersecurity is essential now,” he notes.
Mimicking the human brain: neuromorphic computing
If quantum computing is still some way off production, neuromorphic computing is closer to deployment.
Neuromorphic computing sets out to copy the processes of the human brain in a “spiking neural network”. Existing neural networks, widely used in AI, operate with a constant flow of information. Spiking neural networks, or SNNs, send data in short bursts and add timing to their operations, in a manner closer to the human brain. This makes them more efficient and potentially, more powerful.
The British defense and engineering giant BAE Systems has flagged neuromorphic computing as one of the trends to watch over the coming year.
“Neuromorphic computing will become increasingly important in 2025 and beyond, particularly as AI demands more from computing than ever before,” Rob Wythe, the company’s chief technologist, wrote in BAE’s 2025 technology predictions.
Wythe suggests that SNNs could make AI decisions more defensible, because neuromorphic systems organise themselves into patterns which are easier to understand than the “black box” approach of current AI decisions. And neuromorphic systems could find a role in AI at the edge, where access to vast systems running LLMs is not an option.
“Neuromorphic chips can provide an answer here, by delivering a low power and highly constrained weight, size, power and cost environment,” notes Wythe.
It is easy to see why this would be useful for a defence and aerospace manufacturer such as BAE, given the growing interest in AI systems for military applications such as autonomous weapons systems. But there are wider applications too.
Edge AI is highlighted as a potential use for neuromorphic computing by researchers at IBM. They see its lower power consumption as a way to put AI into devices from smart phones to drones, and in IoT hardware. IBM also sees the technology being used in autonomous vehicles, robotics, and even cybersecurity.
RELATED WHITEPAPER
As Wythe hinted, neuromorphic computing could also unlock far more energy efficient computation, similar to the incredible energy efficiency of the human brain. The US National Institute of Standards and Technology (NIST) states that our brains can perform the equivalent of an exaflop using just 20W.
But, as with quantum technology, neuromorphic systems are not yet available. IBM cautions that standards, programming languages and APIs for neuromorphic systems still need to evolve.
At BAE, Wythe anticipates neuromorphic technology shipping as a hybrid architecture. Systems will have dedicated neuromorphic (or quantum) chips or cards, each playing to their own strength, rather as current systems make use of both CPUs and GPUs. Even so, it is not too soon for CIOs and CTOs to start assessing their potential.
-
Bigger salaries, more burnout: Is the CISO role in crisis?
In-depth CISOs are more stressed than ever before – but why is this and what can be done?
By Kate O'Flaherty Published
-
Cheap cyber crime kits can be bought on the dark web for less than $25
News Research from NordVPN shows phishing kits are now widely available on the dark web and via messaging apps like Telegram, and are often selling for less than $25.
By Emma Woollacott Published